Original Data
Rev Diabet Stud,
2013,
10(4):283-292 |
DOI 10.1900/RDS.2013.10.283 |
Evaluation of the Finnish Diabetes Risk Score (FINDRISC) as a Screening Tool for the Metabolic Syndrome
Mohsen Janghorbani1,2, Hoseinali Adineh2, Masoud Amini1
1Isfahan Endocrine and Metabolism Research Center, Isfahan University of Medical Sciences, Isfahan, Iran
2Department of Epidemiology and Biostatistics, School of Public Health, Isfahan University of Medical Sciences, Isfahan, Iran
Address correspondence to: Mohsen Janghorbani, PhD, Department of Epidemiology and Biostatistics, School of Public Health, Isfahan University of Medical Sciences, Isfahan, Iran, e-mail: janghorbani@hlth.mui.ac.ir
Manuscript submitted January 10, 2014; resubmitted January 20, 2014; accepted February 2, 2014.
Keywords: metabolic syndrome, type 2 diabetes, first-degree relatives, risk score, glucose tolerance
Abstract
OBJECTIVES: Traditionally, the Finnish Diabetes Risk Score (FINDRISC) questionnaire is a screening tool to estimate risk of type 2 diabetes. In this study, we evaluated the ability of FINDRISC to predict the development of the metabolic syndrome (MetS) in an Iranian population without diabetes and MetS. METHODS: A total of 1,010 first-degree relatives of consecutive patients with type 2 diabetes, 30-70 years old (274 men and 736 women), without diabetes and MetS, were examined and followed up over 8.0 ± 1.6 years (mean ± SD) for MetS incidence. The incidence of MetS was examined across quartiles of FINDRISC, and a receiver operating characteristic (ROC) curve was plotted to assess the discrimination. At baseline and through follow-ups, participants underwent a standard 75 g 2-hour oral glucose tolerance test (OGTT). Data for determining FINDRISC were available from each participant. RESULTS: During 8,089 person-years of follow-up, 69 men and 209 women without MetS and diabetes at baseline subsequently developed MetS. The incidence of MetS was 31.4 per 1000 person-years in men and 35.5 in women. The FINDRSC at baseline was significantly associated with MetS evolution. Participants in the top quartile of FINDRISC were 4.4 times more likely to develop MetS than those in the bottom quartile (rate ratio 4.4; 95% CI 2.7-7.0). The area under the ROC curve was 65.0% (95% CI 61.3-68.7). CONCLUSION: The results of this study suggest that FINDRISC can be applied to detect MetS in a high-risk population.
Abbreviations: BMI - body mass index; BP - blood pressure; CI - confidence interval; FINDRISC - Finnish Diabetes Risk Score; FPG - fasting plasma glucose; HbA1c - glycosylated hemoglobin; HC - hip circumference; HDL - high density lipoprotein; IDPS - Isfahan Diabetes Prevention Study; IFG - impaired fasting glucose; IGT - impaired glucose tolerance; LDL - low density lipoprotein; LR - likelihood ratio; MetS - metabolic syndrome; NCEP-ATP III - National Cholesterol Education Program Adult Treatment Panel III; NGT - normal glucose tolerance; NPV - negative predictive value; OGTT - oral glucose tolerance test; PG - plasma glucose; PPV - positive predictive value; ROC - receiver operating characteristic; RR - rate ratio; SD - standard deviation; WC - waist circumference; WHR - waist-to-hip ratio
Introduction
Metabolic syndrome (MetS) constitutes a cluster of metabolic risk factors for cardiovascular disease. It is associated with insulin resistance, which is an important determinant of cardiovascular risk [1]. It is estimated that about a quarter of the world's adult population has MetS [2, 3], and that they are twice as likely to die from and three times as likely to have a heart attack or stroke compared with people without MetS [4]. People with MetS have a fivefold higher risk of developing type 2 diabetes [5]. Thus, living with MetS means a significantly reduced quality of life. The causes for the development of the syndrome remain unclear, but the pathophysiology seems to be largely attributable to insulin resistance, excessive flux of fatty acids, and a chronic proinflammatory state [6].
There is no specific treatment for MetS. Therapy includes lifestyle changes and pharmaceutical agents, but prevention would be preferable. Current evidence suggests that MetS can be prevented through pharmaceutical and lifestyle interventions in high-risk individuals [7], in whom beneficial changes in dietary and exercise behavior have been associated with reductions in several risk factors for cardiovascular disease and diabetes. Thus, given the strong evidence that pharmaceutical and lifestyle interventions prevent MetS, there is much interest in the identification of individuals at high risk of developing MetS, so that preventive action aimed at reducing their risk can be offered.
Early detection of MetS is difficult and population screening, using five clinical and biochemical parameters, would not be practicable or cost-effective, especially in low-income countries. We need a simple, non-invasive, effective tool that uses readily available clinical information for rapid identification of individuals at risk of MetS for use by the general public and in primary health care. These individuals could then be referred to further clinical testing to rule out or diagnose MetS.
The validated Finnish Diabetes Risk Score (FINDRISC) has traditionally been used as a predictor of type 2 diabetes. It takes into account the usual clinical characteristics, such as age, body mass index (BMI), waist circumference (WC), physical activity, dietary consumption of fruits, vegetables, and berries, use of antihypertensive medication, history of high blood glucose, and family history of diabetes.
FINDRISC has been successfully implemented as a practical screening instrument to assess diabetes risk and to detect undiagnosed type 2 diabetes in European populations [8-24]. However, it has also become evident that it is not universally applicable among all ethnic groups and populations [16, 18, 25, 26]. Three cross-sectional studies are available on the relationship between FINDRISC and prevalence of MetS [16, 21, 27], but no cohort study has examined MetS incidence using FINDRISC. Moreover, little is known about the relationship of FINDRISC and MetS in first-degree relatives of patients with type 2 diabetes, who are at higher risk of diabetes and MetS than the general population.
The objective of this longitudinal study was to evaluate the ability of FINDRISC to predict the incidence of MetS in an Iranian population without diabetes and MetS.
Subjects and methods
Data collection
The recruitment methods and examination procedures of the Isfahan Diabetes Prevention Study (IDPS) have been described previously [28]. Briefly, IDPS is an ongoing cohort in central Iran to assess the various potential risk factors for diabetes in subjects with a family history of type 2 diabetes (one of the main risk factors for diabetes). Our study sample comprised 3,409 (895 male and 2,514 female) first-degree relatives of patients with type 2 diabetes. All patients attended clinics at the Isfahan Endocrine and Metabolism Research Center, which is affiliated to the Isfahan University of Medical Sciences, Iran.
The study was conducted between 2003 and 2005. All participants were from Isfahan city and adjoining areas. They completed laboratory tests including a standard 75 g 2-h oral glucose tolerance test (OGTT), a questionnaire on their health status and on various potential risk factors for diabetes, and the FINDRISC questionnaire. The participants were examined in follow-up tests according to the standard of medical care in diabetes [29] to update information on demographic, anthropometric, and lifestyle factors and to diagnose diabetes onset. Accordingly, if OGTT was normal at baseline, repeat testing was carried out at 3-year intervals at least. Otherwise, repeat testing was usually carried out annually. Tenets of the current version of the Declaration of Helsinki were followed, institutional ethical committee approval was granted, and an informed consent form was signed by each participant.
Follow-up and identification of MetS
MetS cases were identified according to the consensus criteria released in 2009 [30], which is equivalent to the third report of the National Cholesterol Education Program Adult Treatment Panel III (NCEP-ATP III) [31]. MetS was considered to be present when at least three of the following five characteristics were determined:
1. Central obesity, defined using ethnic-specific cut-points of waist (waist circumference ≥ 102 cm in men and ≥ 88 cm in women)
2. Triglycerides ≥ 150 mg/dl
3. High-density lipoprotein (HDL) cholesterol <40 mg/dl in men and <50 mg/dl in women
4. Blood pressure (BP) ≥ 130/85 mmHg or on antihypertensive medication
5. Raised plasma glucose, defined as fasting plasma glucose (FPG) ≥ 100 mg/dl.
Pregnant women and people with type 1 and type 2 diabetes were excluded. Participants with type 2 diabetes were excluded because the diagnosis of MetS may have additional meaning in individuals with type 2 diabetes who are treated to counteract the high cardiovascular risk. Other than these, individuals who already had MetS or subjects with a history of taking antidiabetic or lipid-lowering compounds were also excluded. Among the 3,409 persons who participated at baseline, 1,340 subjects were excluded because of diagnosis of type 1 or type 2 diabetes, MetS, or a history of taking antidiabetic or lipid-lowering agents at baseline. Another 1,059 had no follow-up, leaving 1,010 participants included in the study.
However, non-attendees had slightly lower:
- Plasma glucose (PG) at 30 min (134.6 mg/dl versus 140.7 mg/dl, p < 0.001)
- PG at 60 min (133.7 mg/dl versus 142.7 mg/dl, p < 0.001)
- PG at 120 min (107.8 mg/dl versus 115.9 mg/dl, p < 0.001)
- FINDRISC (10.6 vs. 11.2, p < 0.001)
On the other hand, non-attendees had higher levels of:
- Cholesterol (196.2 mg/dl versus 190.9 mg/dl, p < 0.001)
- Low-density lipoprotein (LDL) cholesterol (120.3 mg/dl versus 116.7 mg/dl, p < 0.05)
- HDL cholesterol (49.0 mg/dl versus 47.2 mg/dl, p < 0.01)
Also, non-attendees were slightly older (42.8 year versus 42.2 year, p < 0.05).
The 1,010 participants had a mean age of 42.2 (6.5) years (range 30-70). All participants underwent at least one subsequent review during the follow-up period of 8.0 (1.6) years (range 3-10). Attendees at follow-up visits did not differ significantly from non-attendees regarding most baseline characteristics such as: height, weight, BMI, WC, hip circumference (HC), waist-to-hip ratio (WHR), HbA1c, triglycerides, fasting plasma glucose, systolic and diastolic blood pressure, and obesity.
Follow-up and test procedures
Information on age, gender, body size, HbA1c, cholesterol, LDL cholesterol, HDL cholesterol, triglycerides, BP, family, and personal medical history was collected at baseline and through follow-ups. The same methodology was applied for baseline and follow-up studies.
The participants included siblings and children of patients with type 2 diabetes. They reported to the clinics in the morning after an overnight fast. They were asked to abstain from vigorous exercise in the evening and in the morning of their visit. Smokers were encouraged to abstain from smoking in the morning of the investigations.
Firstly, on arrival at the clinic, the information provided by the participants in the questionnaire on family history was verified. Then, with the subjects in light clothing and without shoes, height, weight, WC, and HC were measured using standard apparatus. Weight was measured to the nearest 0.1 kg on a calibrated beam scale. Height, WC, and HC were measured to the nearest 0.5 cm with a measuring tape. The waist was measured midway between the lower rib margin and the iliac-crest at the end of gentle expiration. Hip circumference was measured over the greater trochanters directly over the underwear. Resting BP was measured after the participants had been seated for 10 min with a mercury sphygmomanometer and appropriately sized cuffs, using standard techniques.
FPG was measured with the glucose oxidase method. Participants with FPG ≥200 mg/dl or pharmacological treatment were considered to be persons with diabetes. If FPG was ≥126 mg/dl and <200 mg/dl, a second FPG was measured on another day. If the second FPG was also ≥126 mg/dl, participants were considered to be diabetic. Those with FPG <126 mg/dl underwent a standard OGTT (75 g glucose 2-h) at baseline and follow-up visits. Venous blood was sampled 0, 30, 60, and 120 min after oral glucose administration. Plasma samples were centrifuged and analyzed the same day.
HbA1c (measured by ion-exchange chromatography), total cholesterol, triglycerides, HDL, and LDL (calculated with the Friedewald equation [32] provided total triglycerides did not exceed 400 mg/dl) were recorded. All blood sampling procedures were performed in the central laboratory of the Isfahan Endocrine and Metabolism Research Center using the enzyme-linked method.
Definitions
Based on the OGTT results, participants were assigned to the following categories:
1. Normal glucose tolerance (NGT, FPG below 100 mg/dl and the 2-h plasma glucose (2hPG) <140 mg/dl)
2. Impaired fasting glucose (IFG, FPG in the range of 100 to 126 mg/dl and the 2hPG was <140 mg/dl)
3. Impaired glucose tolerance (IGT, FPG <126 mg/dl, but with 2hPG concentration ≥140 and <200 mg/dl)
4. Diabetes (FPG ≥200 mg/dl or pharmacological treatment, FPG ≥126 and/or 2hPG of ≥200 mg/dl) [33].
Finnish Diabetes Risk Score (FINDRISC)
FINDRISC was computed for each participant using clinical and questionnaire data collected at baseline. The FINDRISC usually comprises the following eight items [6, 9]:
- Age
- Body mass index (BMI, weight (kg) / height squared (m2))
- Waist circumference (WC)
- Physical activity
- Dietary consumption of fruits, vegetables, and berries
- Use of antihypertensive medication
- History of high blood glucose
- Family history of diabetes.
The maximum achievable score is 26. In the current study, a modified version of the FINDRISC was used. In this shortened version, the following variables were omitted because these items did not add much power for the prediction of diabetes risk in previous studies, as suggested in the original publication [9] and in subsequent studies [18, 25, 34]:
- Dietary consumption of fruits, vegetables, and berries
- Physical activity
Thus, the maximum achievable score on the modified FINDRISC was 23. High blood glucose was defined as IFG and/or IGT at baseline. The modified version of the FINDRISC and the prevalence of FINDRISC questionnaire components in men and women are presented in Table 1.
Table
1.
Prevalence of components of the modified version of the Finnish Diabetes Risk Score (FINDRISC) in men and women |
|
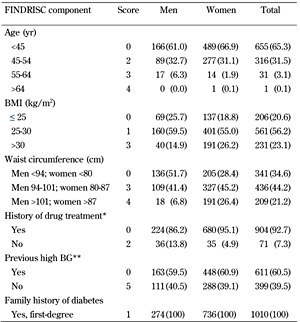 |
 |
Legend:
Data are number (%). The total of each variable may vary because of missing values. * Antihypertensive drug treatment. ** Previously measured high blood glucose. Abbreviations: BG – blood glucose, BMI – body mass index, FINDRISC – Finnish Diabetes Risk Score. |
|
Determination of MetS incidence
The incidence of MetS was expressed as the number of MetS cases per 1000 person-years of follow-up, beginning with the date of completion of the baseline examination in 2003 to 2005 and continuing until the occurrence of MetS, the date of the last completed follow-up, death, or end of follow-up on September 31, 2011, whichever came first.
Statistical analysis
Statistical methods included Student's t-test, chi-squared test, and binary logistic regression. Univariate and multivariate binary logistic regression equations were fitted to identify predictors of new-onset MetS using SPSS version 18 for Windows (SPSS Inc., Chicago, IL, USA).
We calculated the FINDRISC for each participant using baseline age, BMI, WC, use of antihypertensive medication, history of high blood glucose (IFG and/or IGT), and family history of diabetes. We re-coded the FINDRISC into quartile and compared the risk of developing MetS in each quartile with the lowest category of risk (reference group). The ability of FINDRISC, and each component of MetS, to predict the incidence of MetS was examined with receiver operating characteristic (ROC) curves and their respective areas under the curve. Sensitivity was plotted as a function of 1-specificity.
To evaluate the effect of adding components of MetS together, we put each component into a model going from most to least significant as follows:
Model 1: WC and triglyceride
Model 2: WC, triglyceride, and systolic blood pressure
Model 3: WC, triglyceride, systolic and diastolic blood pressure
Model 4: WC, triglyceride, systolic and diastolic blood pressure, and FPG
Model 5: WC, triglyceride, systolic and diastolic blood pressure, FPG, and HDL
The area under the ROC curve is a global summary statistic of the discriminative value of a model, describing the probability that the score is higher in an individual developing MetS than in an individual not developing MetS. Areas under the ROC curves were compared by the algorithm developed by DeLong et al. [35]. All tests for statistical significance were two-tailed, and all tests were performed assuming a type I error probability of <0.05.
Results
Baseline characteristics of the 732 (72.5%) participants without and 278 (27.5%) with MetS were as expected. Those participants who developed MetS were older and had higher mean weight, BMI, WC, HC, WHR, FPG, and PG at 30, 60, and 120 min, higher HbA1c, triglyceride, cholesterol, systolic and diastolic BP, and FINDRISC at baseline (table not shown, can be requested from the authors). A higher proportion of them also had overweight, used antihypertensive medication, and had higher IFG and IGT. The mean (SD) age was 43.3 (6.8) years for those with MetS and 41.8 (6.3) years for those without MetS. The mean (SD) FINDRISC was 12.7 (3.7) for those with MetS and 10.7 (3.7) for those without MetS. The total score ranged from 5 to 21.
The baseline characteristics of the study participants according to FINDRISC quartiles are shown in Table 2. In comparison with variables at baseline, all variables except triglyceride were more likely to increase, height and follow-up duration was more likely to decrease across all four subject groups.
Table
2.
Characteristics of first-degree relatives of patients with type 2 diabetes according to FINDRISC quartiles in the Isfahan Diabetes Prevention Study |
|
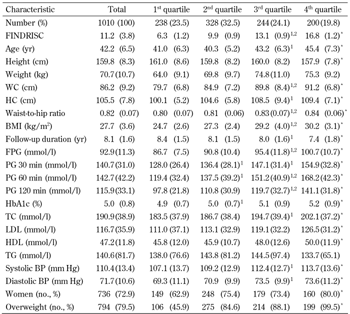 |
 |
Legend:
Data are mean (SD) or number (%). FINDRISC values at baseline. 1st quartile: ≤8.0; 2nd quartile: 8.1-11.0; 3rd quartile: 11.1-14.0; 4th quartile: 14.1-23.0. * p < 0.001, comparison across all four groups. Difference in the mean value of variables compared to the 1 1st quartile and 2 2nd quartile. Abbreviations: BMI – body mass index, BP – blood pressure, CI – confidence interval, FG – fasting glucose, PG – plasma glucose, FINDRISC – Finnish Diabetes Risk Score, HDL – high-density lipoprotein, LDL – low-density lipoprotein, IFG – impaired fasting glucose, IGT – impaired glucose tolerance, NGT – normal glucose tolerance, TC – total cholesterol. |
|
278 incident cases of MetS (27.5%, 69 men and 209 women) occurred during 8,089 (2,197 men and 5,892 women) person-years of follow-up. The overall incidence of subsequent MetS development was 34.3 (95% CI: 30.4-38.3) per 1000 person-years. The incidence rates were slightly higher in women (35.5, 95% CI: 30.7-40.2 per 1000 person-years) than in men (31.4, 95% CI: 24.5-39.6), but the difference was not statistically significant.
The FINDRISC was associated with MetS incidence. The incidence of MetS was 58.7 per 1000 person-years (95% CI 47.3-71.8) for participants in the highest quartile of FINDRISC, and 16.5 per 1000 person-years (95% CI 11.4-23.1) for the lowest quartile. The risk of MetS increased with increasing quartiles of FINDRISC. Compared with participants in the lowest quartile, the risk of MetS was:
1. 4.8 times higher for those in the highest quartile at baseline (rate ratio (RR) 4.8, 95% CI: 3.01-7.59)
2. 3.1 times higher for those in the 3rd quartile (RR 3.1, 95% CI: 2.00-4.95)
3. 1.9 times higher in those in the 2nd quartile (RR 1.9, 95% CI: 1.20-2.93) in unadjusted models
Controlling for age and gender did not appreciably alter the RR compared with the unadjusted model (Table 3).
Table
3.
Incidence rates and rate ratios (RR) of the metabolic syndrome according to FINDRISC quartiles at baseline in the Isfahan Diabetes Prevention Study |
|
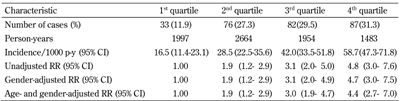 |
 |
Legend:
Data are FINDRISC values at baseline. 1st quartile: ≤8.0; 2nd quartile: 8.1-11.0; 3rd quartile: 11.1-14.0; 4th quartile: 14.1-23.0. Rate ratio (95% CI) calculated by binary logistic regression. Abbreviations: CI – confidence interval, FINDRISC – Finnish Diabetes Risk Score, p-y – person-years, RR – rate ratio. |
|
The ROC curves for the incidence of MetS by FINDRISC, and each component of MetS, are shown in Figure 1. The areas under the ROC curves from the greatest to the least area were:
- 0.650 (95% CI: 0.611-0.689) for FINDRISC
- 0.620 (95% CI: 0.580-0.659) for WC
- 0.588 (95% CI: 0.546-0.630) for triglyceride
- 0.572 (95% CI: 0.531-0.613) for systolic and 0.565 (95% CI: 0.522-0.608) for diastolic BP
- 0.550 (95% CI: 0.507-0.593) for FPG
- 0.542 (0.499-0.585) for HDL
All parameters, except HDL, were significant predictors for future risk of MetS (p < 0.001). Although WC, FPG, triglyceride, HDL, and BP had areas smaller than that of FINDRISC, there were no statistically significances between the areas under the ROC curves for FINDRISC, WC, and triglyceride. The area for FPG, HDL, and systolic and diastolic BP was significantly smaller than that for FINDRISC (p < 0.05).
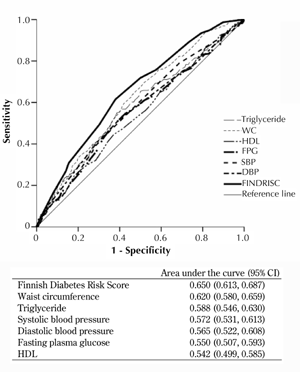 |
 |
Figure 1. Receiver operating characteristic (ROC) curves for FINDRISC, triglyceride, waist circumference (WC), high-density lipoprotein cholesterol (HDL), fasting plasma glucose (FPG), systolic (SBP) and diastolic blood pressure (DBP) to predict metabolic syndrome in first-degree relatives of patients with type 2 diabetes without diabetes or metabolic syndrome at baseline. The estimates of the area under the ROC curves and their 95% CI are shown. |
|
Test characteristics for various FINDRISC cut-off values are presented in Table 4. At a score of 12 or higher, the sum of sensitivity and specificity was maximal. Accordingly, the optimal cut-point for detecting MetS was a FINDRISC greater than or equal to 12. At a FINDRISC greater than or equal to 12, sensitivity was 60.8% and specificity was 62.4%. The corresponding positive and negative predictive values were 37.0% and 70.8%, respectively.
Table
4.
The predictive performance of different Finnish Diabetes Risk Score (FINDRISC) cut-off values for predicting the metabolic syndrome |
|
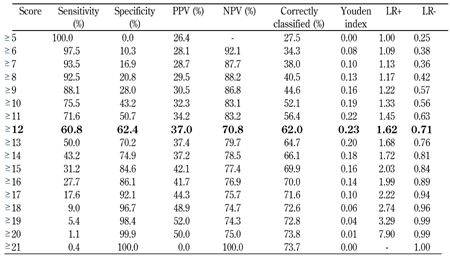 |
 |
Legend:
Values in bold represent best cut-points. Youden index was defined as the maximum of (sensitivity + specificity - 1). Abbreviations: LR+: positive likelihood ratio; LR-: negative likelihood ratio. |
|
The ROC curves for the incidence of MetS by FINDRISC and the models are shown in Figure 2. Adding more components of MetS to the model did not appreciably alter the area under the curve. However there was no significant difference between each model and FINDRISC.
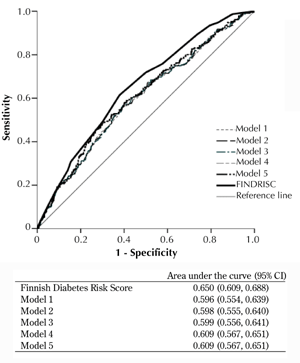 |
 |
Figure 2. Receiver operating characteristic (ROC) curves for FINDRISC, model 1 (WC, triglyceride), model 2 (WC, triglyceride, SBP), model 3 (WC, triglyceride, SBP, and DBP), model 4 (WC, triglyceride, SBP, DBP, and FPG) and model 5 (WC, triglyceride, SBP, DBP, FPG, and HDL) to predict metabolic syndrome in first-degree relatives of patients with type 2 diabetes without diabetes or metabolic syndrome at baseline. The estimates of the area under the ROC curves and their 95% confidence intervals are shown. |
|
Discussion
In this study, the FINDRISC showed a reasonably good ability to predict MetS in a cohort of first-degree relatives of patients with type 2 diabetes, with an area under the ROC of 65%. This was similar to its ability to predict MetS in other populations tested to date [16, 21, 27]. The FINDRISC was originally developed in a prospective setting to identify persons at high risk of developing type 2 diabetes. In this study, we analyzed the score’s performance in a prospective setting as screening tool for the detection of MetS, which is independently associated with future risk of type 2 diabetes [4, 36, 37]. It may therefore be regarded as a useful screening instrument for the identification of high-risk individuals who could benefit from early lifestyle and pharmacological interventions [7, 38].
Although the cause and role of MetS are still controversial, many authors [31, 39] believe that insulin resistance is the 'core aspect' of the syndrome. Under this assumption, it is reasonable to postulate that the factors that contribute most to the development of the MetS should relate most closely to insulin resistance.
The efficiency of risk scores may vary between populations with different ethnic backgrounds. Therefore, risk scores should be validated in each population before use [40, 41]. Although the FINDRISC tool was developed and validated in European populations [8-10, 14-21], it is also valid for Middle-Eastern populations, despite different lifestyles.
Participants in the top quartile of FINDRISC were 4.8 times more likely to progress to MetS than those in the bottom quartile. Those with higher WC at baseline had lower risk of progression to MetS than those with high FINDRISC, further emphasizing the usefulness of FINDRISC in predicting MetS. Thus, the study confirms the reliability of FINDRISC for MetS prediction. FINDRISC may serve as an initial assessment tool to identify persons with a risk of developing type 2 diabetes. The FINDRISC, which uses information routinely available in primary care records, is a simple, inexpensive, and non-invasive tool.
Two cross-sectional studies in Europe and one in Taiwan have assessed the risk of developing MetS by using FINDRISC. Saaristo et al. examined the prevalence of MetS in a Finnish population; the area under the ROC curve for the prevalence of MetS (NCEP-ATP III definition) was 72.4% in men and 75.3% in women [21]. A Greek cross-sectional study found an area under the ROC curve of 70.7% in men and 75.7% in women using a modified version of FINDRISC [16]. A Taiwanese cross-sectional study evaluated a FINDRISC questionnaire, and compared it with 9 other available risk scores for the detection of unknown MetS, demonstrating an area under the ROC of 77.0% using FINDRISC [27]. The area under the ROC curve for the prevalence of MetS in all these studies was higher than that in the present study. However, the other studies could only provide information on the present condition of the tested individuals as they all had a cross-sectional design.
Our study has several strengths and limitations. The strengths include the use of a sample consisting of both men and women, performance of standard OGTT, and information on potential determinants of MetS. There was unlikely to be selection or information bias because of the prospective design. At follow-up, non-attendees did not differ from attendees in the entire population according to major risk factors for the development of MetS, although a difference too small to explain the high progression rate to MetS in our study was seen in the mean levels of lipid profiles, PG, and FINDRISC.
Our database is one of the few that followed first-degree relatives of patients with type 2 diabetes, thereby enabling us simultaneously to control the genetic factors that may predict insulin resistance status. Our study was limited to a cohort of individuals who are at increased risk of developing type 2 diabetes, because they were first-degree relatives of patients with type 2 diabetes. Therefore, a possible selection bias is more likely to underestimate associations than to exaggerate them.
The length of follow-up in the IDPS cohort was relatively short. Thus, while the study proved effective in identifying first-degree relatives of patients with type 2 diabetes who rapidly progress to MetS, those with slower disease onset may not have been identified. Alternatively, longer follow-up periods may increase the RR for the association between FINDRISC and the incidence of MetS if more people in the highest risk score category go on to develop MetS. Therefore, it is necessary to validate the association of FINDRISC and MetS in other populations.
This study evaluated the modified FINDRISC instead of the full risk model, which also includes daily consumption of vegetables, fruits, and berries, and physical activity [10]. As our information on these two risk factors was insufficient we excluded them. Both factors are considered to be important and were primarily targeted during recent diabetes prevention trials. However, in the Lindstrom and Tuomilehto model, both daily intake of vegetables and fruits or berries and physical inactivity were not statistically significant. They were included in the model mainly because prevention studies have demonstrated their importance [10, 42, 43]. It is difficult to apply simple variables for such complex behavioral patterns such as physical activity and diet. In terms of the definition of MetS incidence used, a selection bias may be present as participants who attend for screening may have been more likely to be tested. Consequently, they have been diagnosed with MetS. On the other hand, participants with MetS who had a low risk score may not have been identified because they had not been tested.
Conclusion
In conclusion, our data provide further evidence that FINDRISC can be a suitable tool to predict MetS in a high-risk population and to identify undetected MetS in clinical practice.
Author contributions: MJ conceived and designed the study, analyzed the data, and wrote the manuscript. HA contributed to data analysis and revised the manuscript. MA recruited samples and contributed to the discussion and revision of the manuscript.
Disclosures/Conflict of Interests: The authors report no conflict of interests.
Acknowledgments:
We are grateful to Mr. Abyar for technical computer assistance. This study could not have been conducted without the contributions of the relatives of patients with type 2 diabetes to whom our deep thanks are due.
References
- Lakka HM, Laaksonen DE, Lakka TA, Niskanen LK, Kumpusalo E, Tuomilehto J, Salonen JT. The metabolic syndrome and total and cardiovascular disease mortality in middle-aged men. JAMA 2002. 288:2709-2716. [DOD] [CrossRef]
- Ford ES, Giles WH, Dietz WH. Prevalence of the metabolic syndrome among US adults: findings from the Third National Health and Nutrition Examination Survey. JAMA 2002. 287:356-359. [DOD] [CrossRef]
- Dunstan DW, Zimmet PZ, Welborn TA, De Courten MP, Cameron AJ, Sicree RA, Dwyer T, Colagiuri S, Jolley D, Knuiman M, Atkins R, Shaw JE. The rising prevalence of diabetes and impaired glucose tolerance: the Australian diabetes, obesity and lifestyle study. Diabetes Care 2002. 25:829-834. [DOD] [CrossRef]
- Isomaa B, Almgren P, Tuomi T, Forsen B, Lahti K, Nissen M, Taskinen MR, Groop L. Cardiovascular morbidity and mortality associated with the metabolic syndrome. Diabetes Care 2001. 24:683-689. [DOD] [CrossRef]
- Stern MP, Williams K, Gonzalez-Villalpando C, Hunt KJ, Haffner SM. Does the metabolic-syndrome improve identification of individuals at risk of type 2 diabetes and/or cardiovascular disease? Diabetes Care 2004. 27:2676-2681. [DOD]
- Eckel RH, Grundy SM, Zimmet PZ. The metabolic syndrome. Lancet 2005. 365:1415-1428. [DOD] [CrossRef]
- Orchard TJ, Temprosa M, Goldberg R, Haffner S, Ratner R, Marcovina S, Fowler S, Diabetes Prevention Program Research Group. The effect of metformin and intensive lifestyle intervention on the metabolic syndrome: the Diabetes Prevention Program randomized trial. Ann Intern Med 2005. 142:611-619. [DOD] [CrossRef]
- Saaristo T, Peltonen M, Keinanen-Kiukaanniemi S, Vanhala M, Saltevo J, Niskanen L, Oksa H, Korpi-Hyövälti E, Tuomilehto J, FIN-D2D Study Group. National type 2 diabetes prevention programme in Finland: FIN-D2D. Int J Circumpolar Health 2007. 66:101-112. [DOD] [CrossRef]
- Schwarz PE, Schwarz J, Schuppenies A, Bornstein SR, Schulze J. Development of a diabetes prevention management program for clinical practice. Public Health Rep 2007. 122:258-226. [DOD]
- Lindstrom J, Tuomilehto J. The diabetes risk score: a practical tool to predict type 2 diabetes risk. Diabetes Care 2003. 26:725-731. [DOD] [CrossRef]
- Costa B, Barrio F, Pinol JL, Cabre JJ, Mundet X, Sagarra R, Salas-Salvado J, Sola-Morales O. Shifting from glucose diagnosis to the new HbA1c diagnosis reduces the capability of the Finnish Diabetes Risk Score (FINDRISC) to screen for glucose abnormalities within a real-life primary healthcare preventive strategy. BMC Med 2013. 11:45. [DOD] [CrossRef]
- Soriguer F, Valdes S, Tapia MJ, Esteva I, Ruiz de Adana MS, Almaraz MC, Morcillo S, Garcia Fuentes E, Rodriguez F, Rojo-Martinez G. Validation of the FINDRISC (FINnish Diabetes RIsk SCore) for prediction of the risk of type 2 diabetes in a population of southern Spain. Pizarra Study. Med Clin (Barc) 2012. 138:371-376. [DOD] [CrossRef]
- Musso G. The Finnish Diabetes Risk Score (FINDRISC) and other non-invasive scores for screening of hepatic steatosis and associated cardiometabolic risk. Ann Med 2011. 43:413-417. [DOD] [CrossRef]
- Tankova T, Chakarova N, Atanassova I, Dakovska L. Evaluation of the Finnish Diabetes Risk Score as a screening tool for impaired fasting glucose, impaired glucose tolerance and undetected diabetes. Diabetes Res Clin Pract 2011. 92:46-52. [DOD] [CrossRef]
- Alssema M, Vistisen D, Heymans MW, Nijpels G, Glümer C, Zimmet PZ, Shaw JE, Eliasson M, Stehouwer CD, Tabak AG, et al. The Evaluation of Screening and Early Detection Strategies for Type 2 Diabetes and Impaired Glucose Tolerance (DETECT-2) update of the Finnish diabetes risk score for prediction of incident type 2 diabetes. Diabetologia 2011. 54:1004-1012. [DOD] [CrossRef]
- Makrilakis K, Liatis S, Grammatikou S, Perrea D, Stathi C, Tsiligros P, Katsilambros N. Validation of the Finnish diabetes risk score (FINDRISC) questionnaire for screening for undiagnosed type 2 diabetes, dysglycaemia and the metabolic syndrome in Greece. Diabetes Metab 2011. 37:144-151. [DOD] [CrossRef]
- Wang J, Stancakova A, Kuusisto J, Laakso M. Identification of undiagnosed type 2 diabetic individuals by the Finnish diabetes risk score and biochemical and genetic markers: a population-based study of 7232 Finnish men. J Clin Endocrinol Metab 2010. 95:3858-3862. [DOD] [CrossRef]
- Schwarz PE, Li J, Reimann M, Schutte AE, Bergmann A, Hanefeld M, Bornstein SR, Schulze J, Tuomilehto J, Lindström J. The Finnish Diabetes Risk Score is associated with insulin resistance and progression towards type 2 diabetes. J Clin Endocrinol Metab 2009. 94:920-926. [DOD] [CrossRef]
- Li J, Bergmann A, Reimann M, Bornstein SR, Schwarz PE. A more simplified Finnish diabetes risk score for opportunistic screening of undiagnosed type 2 diabetes in a German population with a family history of the metabolic syndrome. Horm Metab Res 2009. 41:98-103. [DOD] [CrossRef]
- Bergmann A, Li J, Wang L, Schulze J, Bornstein SR, Schwarz PE. A simplified Finnish diabetes risk score to predict type 2 diabetes risk and disease evolution in a German population. Horm Metab Res 2007. 39:677-682. [DOD] [CrossRef]
- Saaristo T, Peltonen M, Lindström J, Saarikoski L, Sundvall J, Eriksson JG, Tuomilehto J. Cross-sectional evaluation of the Finnish Diabetes Risk Score: a tool to identify undetected type 2 diabetes, abnormal glucose tolerance and metabolic syndrome. Diab Vasc Dis Res 2005. 2:67-72. [DOD] [CrossRef]
- Franciosi M, De Berardis G, Rossi MC, Sacco M, Belfiglio M, Pellegrini F, Tognoni G, Valentini M, Nicolucci A. Use of the diabetes risk score for opportunistic screening of undiagnosed diabetes and impaired glucose tolerance: the IGLOO (Impaired Glucose Tolerance and Long-Term Outcomes Observational) study. Diabetes Care 2005. 28:1187-1194. [DOD] [CrossRef]
- Witte DR, Shipley MJ, Marmot MG, Brunner EJ. Performance of existing risk scores in screening for undiagnosed diabetes: an external validation study. Diabetic Med 2010. 27:46-53. [DOD] [CrossRef]
- Brodovicz KG, Dekker JM, Rijkelijkhuizen JM, Rhodes T, Mari A, Alssema M, Nijpels G, Williams-Herman DE, Girman CJ. The Finnish Diabetes Risk Score is associated with insulin resistance but not reduced beta-cell function, by classical and model-based estimates. Diabetic Med 2011. 28:1078-1081. [DOD] [CrossRef]
- Hippisley-Cox J, Coupland C, Robson J, Sheikh A, Brindle P. Predicting risk of type 2 diabetes in England and Wales: prospective derivation and validation of QDScore. BMJ 2009. 338:b880. [DOD] [CrossRef]
- Schwarz PE, Li J, Lindstrom J, Tuomilehto J. Tools for predicting the risk of type 2 diabetes in daily practice. Horm Metab Res 2009. 41:86-97. [DOD] [CrossRef]
- Lin JW, Chang YC, Li HY, Chien YF, Wu MY, Tsai RY, Hsieh YC, Chen YJ, Hwang JJ, Chuang LM. Cross-sectional validation of diabetes risk scores for predicting diabetes, metabolic syndrome, and chronic kidney disease in Taiwanese. Diabetes Care 2009. 32:2294-2296. [DOD] [CrossRef]
- Amini M, Janghorbani M. Diabetes and impaired glucose regulation in first degree relatives of patients with type 2 diabetes in Isfahan, Iran: Prevalence and risk factors. Rev Diabet Stud 2007. 4:169-176. [DOD] [CrossRef]
- Executive summary: Standard of Medical Care in Diabetes 2008. Diabetes Care 2008. 31:S5-S11. [DOD] [CrossRef]
- Alberti KG, Eckel RH, Grundy SM, Zimmet PZ, Cleeman JI, Donato KA, Fruchart JC, James WP, Loria CM, Smith SC Jr. Harmonizing the metabolic syndrome: a joint interim statement of the International Diabetes Federation Task Force on Epidemiology and Prevention; National Heart, Lung, and Blood Institute; American Heart Association; World Heart Federation; International Atherosclerosis Society; and International Association for the Study of Obesity. Circulation 2009. 120:1640-1645. [DOD] [CrossRef]
- Expert Panel on Detection Evaluation and Treatment of High Blood Cholesterol in Adults. Executive summary of the third report of the National Cholesterol Education Program (NCEP) Expert Panel on Detection Evaluation and Treatment of High Blood Cholesterol in Adults (Adult Treatment Panel III). J Am Med Assoc 2001. 285:2486-2497. [DOD] [CrossRef]
- Friedewald WT, Levy RI, Fredrickson DS. Estimation of the concentration of low-density lipoprotein cholesterol in plasma, without use of the preparative ultracentrifuge. Clin Chem 1972. 18:499-502. [DOD]
- Expert Committee on the Diagnosis and Classification of Diabetes Mellitus. Report of the expert committee on the diagnosis and classification of diabetes mellitus. Diabetes Care 2003. 26(Suppl 1):S5-S20. [DOD]
- Schuppenies A, Jacobey H, Bornstein S, Schwarz PE. FINDRISK-Development of a questionnaire to estimate the risk of diabetes. Ernaehrungsumschau 2006. 53:386. [DOD]
- DeLong ER, DeLong DM, Clarke-Pearson DL. Comparing the areas under two or more correlated receiver operating characteristic curves: a nonparametric approach. Biometrics 1988. 44:837-845. [DOD] [CrossRef]
- Lorenzo C, Okoloise M, Williams K, Stern MP, Haffner SM, San Antonio Heart Study. The metabolic syndrome as predictor of type 2 diabetes: the San Antonio heart study. Diabetes Care 2003. 26:3153-3159. [DOD] [CrossRef]
- Spijkerman AM, Adriaanse MC, Dekker JM, Nijpels G, Stehouwer CD, Bouter LM, Heine RJ. Diabetic patients detected by population-based stepwise screening already have a diabetic cardiovascular risk profile. Diabetes Care 2002. 25:1784-1789. [DOD] [CrossRef]
- Orchard TJ, Temprosa M, Barrett-Connor E, Fowler SE, Goldberg RB, Mather KJ, Marcovina SM, Montez M, Ratner RE, Saudek CD, et al. Long-term effects of the Diabetes Prevention Program interventions on cardiovascular risk factors: a report from the DPP Outcomes Study. Diabetic Med 2013. 30:46-55. [DOD] [CrossRef]
- Ferrannini E, Haffner SM, Mitchell BD, Stern MP. Hyperinsulinaemia: the key feature of a cardiovascular and metabolic syndrome. Diabetologia 1991. 34:416-422. [DOD] [CrossRef]
- Griffin SJ, Little PS, Hales CN, Kinmonth AL, Wareham NJ. Diabetes risk score: towards earlier detection of type 2 diabetes in general practice. Diabetes Metab Res Rev 2000. 16:164-171. [DOD] [CrossRef]
- Hunt K, Williams K, Haffner S, Stem M. Predicting impaired glucose tolerance among individuals with non-diabetic fasting glucose value: The San Antonio Heart Study. Diabetes 2002. 2:SA229. [DOD]
- Simmons RK, Harding AH, Wareham NJ, Griffin SJ. Do simple questions about diet and physical activity help to identify those at risk of type 2 diabetes? Diabetic Med 2007. 24:830-835. [DOD]
- Harding AH, Griffin SJ, Wareham NJ. Population impact of strategies for identifying groups at high risk of type 2 diabetes. Prev Med 2006. 42:364-368. [DOD] [CrossRef]
This article has been cited by other articles:
|
Uptake of screening for type 2 diabetes risk in general dental practice; an exploratory study
Bould K, Scott SE, Dunne S, Asimakopoulou K
Br Dent J 2017. 222(4):293-296
|
|
|
Relationship between the Finnish Diabetes Risk Score (FINDRISC), vitamin D levels, and insulin resistance in obese subjects
Lima-Martinez MM, Arrau C, Jerez S, Paoli M, Gonzalez-Rivas JP, Nieto-Martinez R, Iacobellis G
Prim Care Diabetes 2017. 11(1):94-100
|
|
|
Differentiation of obese patients at moderate or higher Findrisc score based on their atherogenic index
Andreeva-Gateva PA, Konsulova P, Orbetzova M, Georgieva-Nikolova R, Tafradjiiska-Hadjiolova R, Angelova V, Voynikov Y, Nikolova I, Simova I
Postgrad Med 2016. 128(8):790-796
|
|
|
Evaluation of the Japanese Metabolic Syndrome Risk Score (JAMRISC): a newly developed questionnaire used as a screening tool for diagnosing metabolic syndrome and insulin resistance in Japan
Tan C, Sasagawa Y, Kamo KI, Kukitsu T, Noda S, Ishikawa K, Yamauchi N, Saikawa T, Noro T, Nakamura H, Takahashi F, Sata F, Tada M, Kokai Y
Environ Health Prev Med 2016. 21(6):470-479
|
|
|
Prevalence of undiagnosed diabetes and prediabetes among voluntary blood donors in a tertiary health care setting
Ramasamy J, Parthasarathy UK
Int J Res Med Sci 2016. 4(7):2569-2575
|
|
|
Retrospective Assessment of Family Medicine Office Patients in Last Three Years
Fidanci I, Eren SÜ, Arslan I, Tekin O
DergiPark 2016. 8(3):151-157
|
|
|