Original Data
Rev Diabet Stud,
2016,
13(4):246-256 |
DOI 10.1900/RDS.2016.13.246 |
Dietary Patterns and 10-year (2002-2012) Incidence of Type 2 Diabetes: Results from the ATTICA Cohort Study
Efi Koloverou1, Demosthenes B. Panagiotakos1, Ekavi N. Georgousopoulou1, Athanasios Grekas1, Aimilia Christou1, Michail Chatzigeorgiou1, Christina Chrysohoou2, Dimitrios Tousoulis2, Christodoulos Stefanadis2, Christos Pitsavos2, ATTICA Study Group
1Department of Nutrition and Dietetics, School of Health Science and Education, Harokopio University, Athens, Greece
2First Cardiology Clinic, School of Medicine, University of Athens, Greece
Address correspondence to: Demosthenes B. Panagiotakos, Department of Nutrition and Dietetics, School of Health Science and Education, 70 Eleftheriou Venizelou Ave., 17671 Athens, Greece, e-mail: dbpanag@hua.gr
Manuscript submitted December 26, 2016; resubmitted January 11, 2017; accepted January 31, 2017.
Keywords: type 2 diabetes, Mediterranean diet, dietary pattern, prediabetes, diabetes incidence, factor analysis
Abstract
AIM: To identify dietary patterns among apparently healthy individuals and to determine their long-term effect on diabetes incidence. METHODS: During 2001-2002, a random sample of 3,042 men and women (18-89 years old), living in greater Athens, was randomly selected to participate in the study. During 2011-2012, the 10-year follow-up was performed in 2,583 participants (15% drop-out rate). After excluding participants with diabetes at baseline and those for whom no information on diabetes status was available at follow-up, the working sample consisted of 1,485 participants. Dietary habits were assessed by means of a validated semi-quantitative, food frequency questionnaire. Factor analysis was performed to extract dietary patterns from 18 food groups. RESULTS: Diabetes diagnosis at follow-up was made in 191 participants, yielding an incidence rate of 12.9%. Six factors (i.e. dietary patterns) were identified that explained 54% of the variation in consumption. After adjusting for major confounders, and stratification by age-group, logistic regression revealed that the most healthful pattern consisted of the consumption of fruits, vegetables, legumes, bread, rusk, and pasta which reduced the 10-year diabetes risk by 40%, among participants aged 45-55 years. The association reached marginal statistical significance (95% CI: 0.34, 1.07), while no significant association was observed for the other age-groups. When the analysis was additionally adjusted for carbohydrate percentage, statistical significance was lost completely, suggesting a possibly mediating effect of this macronutrient. CONCLUSIONS: The results confirm the potentially protective effect of a plant-based dietary pattern in the primary prevention of diabetes, in particular among middle-aged people. Carbohydrate content may be a specific factor in this relationship; other micronutrients found in plant-based food groups may also play a role.
Abbreviations: BMI - body mass index; CVD - cardiovascular disease; CI - confidence interval; FFQ - food-frequency questionnaire; HDL - high-density lipoprotein; IPAQ - International Physical Activity Questionnaire; LDL - low-density lipoprotein; OR - odds ratio; WC - waist circumference
1. Introduction
The World Health Day 2016 campaign was dedicated to the devastating impacts and consequences of type 2 diabetes which has reached epidemic dimensions. The World Health Organization reported that, in 2014, 9% of the adult population was diagnosed with diabetes; it is estimated that the disease will rise to the 7th place among the leading diseases by 2030 [1, 2]. Patients face a 2-4 times higher risk of cardiovascular disease (CVD) or death from any cause associated with the disease [3]. These alarming figures underlie the need for effective therapeutic means and preventive strategies, especially in middle- and low-income countries.
Genetic susceptibility is an important factor in the pathogenesis of diabetes, but acts mainly as a trigger in relation to specific environmental factors. Therefore, weight management and regular physical activity are common targets of interventional programs as they have been inversely associated with diabetes development and progression [4]. Although diet has long been recognized as a therapeutic tool in effective diabetes management [5], its role on primary disease prevention still remains unclear. The effects of specific food groups on the onset of diabetes and its complications have been studied extensively. Several food groups such as whole grain, low-fat dairy products, fruits, vegetables, pulses, and nuts (in women) have been found to be correlated positively with reduced risk of diabetes, while sugar-rich beverages, meat, processed meat, hydrogenated oils, margarines, and eggs seem to increase the risk of diabetes [6]. In recent times, the use of the patterns approach has shown advantages over the consideration of separate food groups, for both practical and methodological reasons [7]:
- Foods may have synergistic or competing properties, which may alter the true food-health relationship.
- Individuals consume a variety of food combinations. Therefore, eating patterns reflect dietary habits more accurately.
- A pattern is more easily translatable into guidelines in everyday clinical practice, compared to the intake of specific nutrients.
Nutritional epidemiology has used the following two statistical approaches for the identification of dietary patterns:
- A-priori method (based on an already described pattern)
- A-posteriori method (based on a study describing a new pattern that has been derived empirically) [8]
Various studies have used a-priori methods to evaluate the relation between adherence to a dietary pattern and diabetes pathogenesis among initially healthy individuals. The most common pattern tested is the Mediterranean diet, which has consistently been found to lower diabetes risk [9-11]. The pattern is characterized by:
- Abundant consumption of plant foods and olive oil as the principal source of fat.
- Moderate consumption of fish, poultry, eggs, dairy products, and alcohol.
- Low consumption of meat, its products, processed foods, and sugar [12].
Some studies have used a-posteriori methods to find new dietary patterns, and evaluate their long-term effects on the risk of diabetes [12-14]. Irrespective of the method applied, the results of studies on the relation of diet to risk of diabetes are inconsistent. A meta-analysis of 10 prospective studies, in which Caucasians, Africans, Hispanics, and Chinese were equally represented, concluded that "healthful" patterns, consisting of fruits, vegetables, and whole grain products, may reduce diabetes risk by 14%, whereas "unhealthful" patterns, including high contents of red and processed meat, high-fat dairy, and refined grains, may increase the risk by 30% [15]. However, a very recent case-control study did not find a significant association between healthful dietary patterns and diabetes risk [16]. Furthermore, most studies have focused on subjects aged over 40 years. This means that data on younger subjects are lacking, which is particularly relevant in type 2 diabetes as patients are becoming increasingly younger. To the best of our knowledge, no study has investigated the potential differentiated effect of a dietary pattern by age group until today. Finally, no relevant study has been conducted in Greece, a country that experienced low CVD rates in the 1960s and 1970s, but has high diabetes rates today, with 13 new cases per 1000 adults per year [17], which is consistent with the global trend.
Therefore, in the present study, we investigated the effects of dietary patterns on the 10-year incidence of diabetes in a large, representative sample of CVD-free Greek adults, aged over 18 years.
2. Methods
2.1 Baseline sampling procedure (2001-2002)
The ATTICA study is a large-scale, prospective, health- and nutrition-related survey. The baseline sampling of participant data was conducted during 2001-2002 in the province of Attica, where Athens is the major metropolis. In each area, the information was collected during all four seasons to eliminate the potential confounding effect of seasonality on dietary habits. Out of the 4,056 inhabitants of the above area invited initially, and after excluding those with CVD (i.e., n = 117) and those with chronic viral infections (n = 107), 3,042 individuals agreed to participate (75% participation rate); 1,514 of the participants were men (aged 46 ± 13 yr; range 18-87 yr) and 1,528 were women (aged 45 ± 13 yr; range: 18-89 yr). Trained personnel (i.e., cardiologists, general practitioners, dietitians, and nurses) interviewed the participants following a standard methodology. The study was conducted according to the Declaration of Helsinki guidelines; all procedures involving human subjects were approved by the ethics committee of the First Cardiology Department of the University of Athens. Written informed consent was obtained from all individuals. General information about the aims, design, and methods of the ATTICA study may be found elsewhere [18].
2.2 Baseline measurements (2001-2002) - dietary assessment
The evaluation of the dietary habits was based on a validated, semi-quantitative food-frequency questionnaire (FFQ), the EPIC-Greek questionnaire, which was kindly provided by the Unit of Nutrition from the Athens Medical School [19]. The participants were instructed by trained personnel to complete the questionnaire, which included 156 foods and beverages commonly used in Greece. In this work, we focused on major food groups, including dairy products (milk, yogurt, and cheese), fruits, vegetables, legumes, starchy foods (cereals, bread, pasta, potatoes, and rice), meat (red, white, and processed), small and big fish, eggs, nuts, and sweets. Beverages such as alcohol, coffee, soda drinks, and tea were not considered. Added fat was recorded, but also not considered in the analysis since the majority of participants (about 95%) reported the use of olive oil as the main fat added, causing this variable to be almost constant. In this population, the exclusive use of olive oil as added oil and in cooking is a tradition carried on until today.
2.3 Socio-demographic and other lifestyle variables
Information about socio-demographic characteristics (age, sex, and years of school), personal and family history of hypertension, hypercholesterolemia and diabetes, family history of CVD, smoking status, and physical activity was collected. Smokers were defined as persons who smoked at least one cigarette per day or who had quitted within the previous year; the rest were defined as non-smokers. The International Physical Activity Questionnaire (IPAQ), an index of weekly energy expenditure that records the frequency (times/week), duration (in minutes), and intensity of physical activity (in expended calories per time), was used to determine the level of physical activity [20].
2.4 Anthropometric, clinical, and biochemical characteristics
Weight (in kg), height (in m), waist and hip circumference (in cm), and clinical characteristics were measured using standard procedures. Arterial blood pressure was measured 3 times using the right arm. All measurements were performed at the end of the physical examination, while subjects were in a sitting position for at least 30 min. When average blood pressure was ≥140/90 mm Hg or when antihypertensive medication was used, the participant was classified as hypertensive. Blood samples were collected from the antecubital vein between 8 to 10 a.m., in a sitting position after 12 hours of fasting and alcohol abstinence. Serum total cholesterol, HDL-cholesterol, triglycerides, and glucose concentrations were measured using the chromatographic enzymatic method by a Technicon automatic analyzer RA-1000. LDL cholesterol was calculated using the Friedewald formulae. Hypercholesterolemia was defined as total serum cholesterol concentrations >200 mg/dl or the use of lipid-lowering agents. Serum insulin concentrations were assayed by means of a radioimmunoassay. Diagnosis of type 2 diabetes was conducted according to American Diabetes Association criteria [21], i.e., fasting blood glucose >125 mg/dl or the use of antidiabetic agents and/or insulin.
2.5 Ten-year follow-up evaluation (2011-2012)
The 10-year follow-up was performed during 2011-2012. Of the 3,042 participants, 2,583 completed the follow-up (85% participation rate). A detailed evaluation of the participants' medical status was performed. Among various endpoints, development of diabetes mellitus was recorded. Patients who had diabetes at baseline (n = 210), and those for whom information about diabetes status was not available at the 10-year follow up (n = 888), were excluded from the present analyses, yielding a working sample of n = 1,485 participants. The sample size was adequate to achieve 92% statistical power for the evaluation of a relative risk of 0.70 between the null and the alternative two-sided hypothesis, when the exposure variable was increased by 1 unit and with a significance level (alpha) of 0.05. Further details about the baseline and 10-year follow-up procedures of the study are described elsewhere [17, 18].
2.6 Statistics - food factor derivation
Factor analysis, using the principal component method, was applied to identify dietary patterns that show protection against diabetes pathogenesis. The correlation matrix of the food variables used here showed that there were several correlation coefficients with r >0.4. Moreover, the phi coefficient (a measure of the inter-association of variables) was 0.89, which is close to 1. Both approaches implied high interrelationships between the food variables. Therefore, factor analysis was effective for assessing the food patterns.
From the entire food database derived from the FFQ (see subsection 2.3), 18 food categories were selected to represent all major food groups, i.e., dairy products, fruits, vegetables, legumes, cereals, etc. (Table 1). Food groups that entered the analysis were coded as servings per month. The orthogonal rotation (rotation with varimax option) was used to derive optimal non-correlated factors. The factors are dietary patterns that are characterized by the predominant consumption of specific food groups, e.g. red or white meat and potatoes for one factor. The information was rotated to increase the representation of each food or food group to a factor.
Table
1.
List of the 18 foods or food groups selected from the entire food frequency questionnaire database |
|
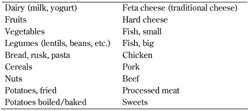 |
 |
Eigenvalues were derived from the correlation matrix of the standardized variables. The eigenvalue is a value that indicates the proportion of the variation in consumption explained by each factor. The scree plot of the eigenvalues was examined to decide the number of factors retained. According to Kaiser's criterion, the factors that should be retained are those with eigenvalues >1. In our analysis, a six-factor solution was selected (six dietary patterns), because six of the 18 extracted factors had eigenvalues >1. We then calculated factor scores, which are interpreted similarly to correlation coefficients, i.e., higher absolute values indicate that the food variable contributes most to the construction of the factor. The patterns were indicated according to the scores of the food groups that correlated most strongly with the factors (scores 0.4).
2.7 Descriptive and basic statistical analyses
The incidence of diabetes was calculated as the ratio of new cases (n = 191) to the total number (n = 1,485) of participants in the follow-up. Continuous variables were indicated as mean values ± standard deviation and categorical variables as frequencies. After ensuring equality of variances using Levene's test, comparisons of the mean values of normally distributed variables between participants who developed diabetes and those who did not were performed using Student's t-test. For non-normally distributed variables, the Mann-Whitney test was applied. Continuous variables were tested for normality by the Kolmogorov-Smirnov test. For categorical variables, the chi-squared test was used. Since the extracted factors (i.e., dietary patterns) were not normally distributed, their correlations with diabetes risk were tested using Spearman's rho test for continuous variables or Mann-Whitney test for categorical variables.
Slightly adjusted, multiple linear regression models were constructed using factor scores as dependent variables and major confounders as independent variables. The results were expressed as b-coefficient ± SE. The risk of developing diabetes during the 10-year study period in relation to adherence to a dietary pattern was estimated using odds ratios (OR) in combination with the respective 95% confidence intervals derived from multiple logistic regression. This type of analysis was preferred (instead of survival analysis) as there were no data on diabetes onset, but only diagnosis. Interactions of each confounder with extracted dietary patterns were checked in all steps of the analysis. Known confounders were included in the models after testing for collinearity. The significance level (alpha) of 0.05 was used, and all reported p-values were based on two-sided tests. SPSS software (Statistical Package for Social Sciences, IBM Hellas SA, Greece, version 18) was used for all statistical calculations.
3. Results
3.1 10-year diabetes incidence
During the 10-year follow-up period, 191 diabetes cases were recorded, yielding a crude incidence of 129 per 1000 participants (or 12.9%). Of the 191 cases, 97 (13.4%) were men and 94 (12.4%) were women (p = 0.79 for gender difference) [17].
3.2 Participants' baseline characteristics by diabetes status at 10-year follow-up
Demographic and clinical characteristics of the ATTICA study participants by diabetes status at 10-year follow-up are presented in Table 2. Unadjusted analysis revealed that, at baseline, participants who developed diabetes were older by almost a decade, were more likely to be predisposed to diabetes, had higher fasting glucose levels, and a greater proportion had hypertension, hypercholesterolemia, and the metabolic syndrome, compared to those that did not develop diabetes. They also had higher initial body mass index (BMI) and waist circumference, and were more likely to have an abnormal waist-to-hip ratio. Smoking status, years of education, energy intake, and physical activity did not differ significantly between the two groups at baseline.
Table
2.
Baseline characteristics of the ATTICA study participants according to 10-year diabetes incidence (n = 1,485) |
|
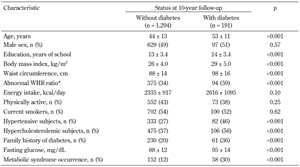 |
 |
Legend:
Data are presented as mean values and standard deviation or absolute and relative frequencies. P-values were derived from Student's t-test for the normally distributed variables and Mann-Whitney test for the non-normally distributed variables (i.e., years of school, fasting glucose), or chi-square test for the categorical variables. * >0.8 for women and >1.0 for men. |
|
Slight differences in certain baseline characteristics were noted between those for whom information about 10-year diabetes status was available (n = 1,485) and those for whom it was not (n = 1,347). These characteristics concerned the distribution of age (43 ± 13 vs. 45 ± 13 years, p < 0.001), hypertension (30% vs. 26%, p = 0.036), smoking status (58% vs. 54%, p = 0.028), abnormal waist circumference (50% vs. 54%, p = 0.027), and fasting glucose level (88 ± 12 vs. 80 ± 13, p = 0.005). No differences were found in the distribution of sex, years of education, family history of diabetes, physical activity, metabolic syndrome, hypercholesterolemia, BMI, abnormal waist-to-hip ratio, and energy intake (all p > 0.05).
3.3 Dietary patterns
Factor analysis identified six factors (dietary patterns) that explained 54% of the total variation in consumption. The scores for the six factors are presented in Table 3 (the coefficients with absolute value greater than 0.4 are presented in bold). As mentioned previously, the higher the absolute value of a specific food or food group the higher is its participation in the development of the factor. We have derived the following six factors (dietary patterns) that were characterized by predominant consumption of the following food groups:
Factor 1: Red or white meat (beef, pork, and poultry) and potatoes (fried, boiled, or baked)
Factor 2: Fruits, vegetables, legumes, bread, pasta, rusk (the healthful pattern)
Factor 3: Processed meat and all kind of cheese
Factor 4: Fish (small and big)
Factor 5: Nuts and sweets
Factor 6: Dairy and cereals
Table
3.
Factor coefficient for foods or food groups consumed by the Greek ATTICA study participants (n = 1,485) at baseline |
|
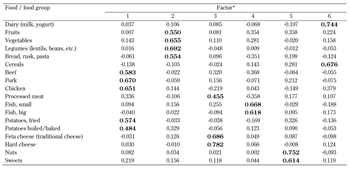 |
 |
Legend:
Score coefficients were similar to the correlation coefficient, with higher absolute values indicative of higher correlation between the (food) variable and the respective factor. * The patterns were predominantly characterized by the consumption of meat and potatoes (Factor 1), fruits, vegetables, legumes, bread, rusk, and pasta (Factor 2), processed meat and cheese (Factor 3), fish (Factor 4), nuts and sweets (Factor 5), dairy and cereals (Factor 6). |
|
Interestingly, some food groups were not as inter-correlated as expected, e.g., cheese was not correlated with milk and yogurt, and poultry was not correlated with fish. This led to the classification of these food products into different food patterns. Factor 1 was the most dominant pattern and explained 15.5% of the total variation in consumption. Each of the remaining five factors explained between 5.9% (Factor 6) and 10% (Factor 2) of the variation in consumption.
Correlations between participants' characteristics (i.e., age, sex, years of education, waist circumference, physical activity status, family history of diabetes, smoking, and status of hypertension and hypercholesterolemia) and factor scores were tested (for descriptive reasons). We obtained the following results for the different factors:
- Factor 1 was associated inversely with age (rho = -0.23, p < 0.001) and physical activity status (p = 0.018).
- Factor 2 was associated positively with age (rho = 0.194, p < 0.001) and inversely associated with hypertension (p = 0.008) and hypercholesterolemia status (p = 0.049).
- Other factors: there were no correlations between diabetes risk and the other factors because they explained only a small proportion of the total variation in consumption.
Finally, multiple adjusted regression models were constructed, with Factors 1 and 2 as dependent variables, and age, sex, family history of diabetes, waist circumference, physical activity, smoking, and hypertension and hypercholesterolemia status as independent variables. Factor 1 was inversely associated with age only (b = -0.029 ± 0.005, p < 0.001) and Factor 2 positively associated with age (b = 0.016 ± 0.004, p = 0.001) and hypertension status (b = 0.26 ± 0.11, p = 0.021).
3.4 10-year diabetes incidence and dietary patterns
To evaluate the associations between the extracted factors and the 10-year risk of developing type 2 diabetes, logistic regression was performed. The interaction between factor scores and age groups of subjects was statistically significant, so the analysis was continued with stratification by age groups. As the data was stratified, we included only major confounders in the analysis (i.e., sex, family history of diabetes, waist circumference, and smoking status) to avoid over-adjustment.
The results are shown in Table 4. The healthful pattern characterized by the consumption of fruits, vegetables, and starchy foods showed a 40% lower 10-year risk of developing diabetes (OR = 0.60, 95% CI: 0.34, 1.07) for participants aged 45-55 years at baseline. This result also shows that age plays a particular role in the effect, i.e., mid-aged people seem to benefit most from the carbohydrate-rich, vegetarian diet. No other food pattern reached statistical significance. As Factor 2 represented almost all of the carbohydrate-rich foods consumed in Greece (i.e., bread, rusk, pasta, legumes, fruits, and vegetables), we also included the percentage of calories from carbohydrates in the analysis to test whether the marginally protective effect found for the vegetarian, carbohydrate-rich diet is mediated by the high carbohydrate content. This approach led to loss of statistical significance (OR = 0.62, 95% CI: 0.34, 1.13), indicating that there may be a mediating effect caused by carbohydrates.
Table
4.
Results from multiple logistic regression by age-group among the 1,485 participants in the ATTICA study, which evaluated the association between food factors and 10-year diabetes risk |
|
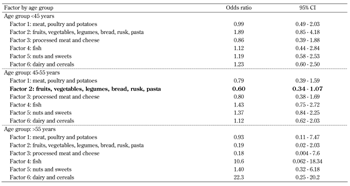 |
 |
Legend:
The analysis was adjusted for major confounders, i.e., gender, family history of diabetes, waist circumference, and smoking status. Since the 1-unit increase in scores for the factors was not meaningful (i.e., 1-unit increase does not mean 1 serving increase) we could only evaluate the direction of the effect size (i.e., hazard ratio >1= "non-protective" or <1= "protective"). |
|
When the model was adjusted for total energy intake (instead of calories by carbohydrates), the marginally significant effect of Factor 2 was lost again (OR = 0.72, 95% CI: 0.35, 1.49), indicating the importance of the total amount of calories consumed on the tested relationship.
4. Discussion
Using factor analysis in a sample of 1,485 adult men and women free of cardiovascular disease, we derived six factors (patterns) which explained 54% of the total variation in dietary habit. Factor 1 was characterized by the consumption of red or white meat and potatoes, a typical Greek eating behavior; it had the largest part of variation in consumption, namely 15.5%. Factor 2 could be described as a healthful pattern, as it featured high-quality, carbohydrate-rich foods, specifically fruits, vegetables, legumes, bread, rusk, and pasta. This pattern explained 10% of the variation in nutrition. The rest of the patterns represented other foods and food groups, including processed meat, cheese, fish, nuts, sweets, dairy products, and cereals, which explained approximately 6-8% of the total variation.
The above-mentioned dietary patterns were studied in relation to 10-year diabetes risk. The analysis showed that age has a significant influence on the impact of diet on diabetes development. After sample stratification by age group, only the healthful pattern was found to decrease significantly the 10-year diabetes risk by 40% among participants aged 45-55 years. The inclusion of the percentage of carbohydrates in the fully adjusted model caused a slight change in the 95% CI, which means that the protective effect of the plant-based pattern (Factor 2) may partially be attributed to their greater carbohydrate and lesser fat content. Of course, the observed antidiabetic effect may be induced by different aspects of the diet, including other micronutrients, antioxidants, fibers, magnesium, etc. present in the food groups as well as potential nutritional interactions. It may be speculated that the high percentage of "high-quality carbohydrates" in the diet leads to a higher intake of antioxidants, magnesium, and fibers (which is linked to decreased diabetes risk), and a smaller intake of saturated and trans fatty acids (which is supposed to increase the risk) [6]. Finally, adjustment for calories had the same effect as the adjustment for carbohydrates, indicating the importance of quantity, i.e. calories consumed (instead of quality), in a dietary pattern.
Despite the observational nature of the present study, the large, representative sample, the prospective design, and the long follow-up period of 10 years in combination with the detailed assessment of lifestyle information, which allowed the adjustment for several known confounders, made the analysis meaningful and robust against overestimation. The finding supports the claim for healthful eating as it has a protective effect against diabetes development. As a new outcome, middle-aged people seem to benefit most from long-term adherence to the healthy eating pattern.
Pattern analysis has emerged as a complementary statistical approach when attempting to investigate the multidimensional essence of nutrition and its relationship with a chronic disease. A diet is more than the sum of its components. The synergistic effects of a diet exceed the combined effects of single food components, an insight that has led to fundamental concerns about food-specific, single-variable approaches. Therefore, studying food patterns may better delineate the variation in proportions, combinations, and frequencies of consumed foods and beverages. Furthermore, traditional, single-variable analysis is afflicted with methodological deficits, because the high level of inter-correlation between food variables makes the estimation of the effect size of singe foods or nutrients in regression models difficult.
As discussed above, there are two methods available to identify food patterns. A-priori analysis is intended to measure the level of adherence to a pre-specified healthful dietary pattern, using diet indexes. On the other hand, a-posteriori analyses (principal component, cluster, or factor) do not share the limitations of a "static" evaluation based on "expert" opinion; rather they generate dietary patterns incorporating characteristics of individual choices in relation to the development of a disease [22]. Previous studies have used the a-posteriori methodology to identify dietary patterns in relation to diabetes pathogenesis.
The present study also followed the a-posteriori method to identify the most healthful pattern, which was a vegetarian-like diet, rich in fruits, vegetables, legumes, and wholegrain cereals, and poor in animal products. Similar patterns have already been described in other studies. Using cluster analysis, Brunner et al. studied 7,731 men and women (mean age 50 years) for 15 years, and detected a healthful pattern that included fruits, vegetables, whole-meal bread, low-fat dairy products, and small amounts of alcohol [8]. Montonen et al. studied 4,303 men (aged 40-69 years) for 23 years, and described a pattern labeled "prudent" that was characterized by increased consumption of fruits and vegetables [23]. Similarly, in a 4-year prospective study, Hodge et al. observed 36,787 adults from the Melbourne Collaborative Cohort, and described a similar pattern that was characterized by higher consumption of salad and cooked vegetables [14]. Finally, in a larger sample consisting of 42,504 men aged 40-75 years, the "prudent" pattern was not only characterized by fruits and vegetables, but also by fish, poultry, and whole grain [13]. In all previous studies, the healthful patterns were associated with a reduced risk of diabetes. Although there is no standard definition of a "healthful pattern", it shows that fruits and vegetables were part of all healthful patterns detected in these studies. Interestingly, a very recent meta-analysis of 10 prospective studies concluded that a reduced risk of 14% may be achieved when adopting a healthful pattern consisting of fruits, vegetables, and whole grains [15]. These results further confirm the existence of a healthful, anti-diabetic vegetarian-based diet.
Another interesting finding from the present study was the effect of age-groups on the tested relationship. The pattern characterized by high amounts of fruits, vegetables, and complex carbohydrates was significantly associated with reduced diabetes risk, but only among middle-aged participants (45-55 years). It is well known that diabetes risk increases with age, with every year adding approximately 14% to the risk, and it has been previously reported that ATTICA study participants aged 45-54 years at baseline belong to the age-group with the most diabetes cases at 10-year follow-up [17]. It may thus be hypothesized that this group of participants benefited most from a healthy diet since this decade is critical for diabetes onset. To the best of our knowledge, no previous study has reported this finding; more studies are necessary to confirm it.
The proposed effect of the healthful pattern against diabetes development may be ascribed to several mechanisms. Following a food approach, this pattern is high in fruits and vegetables, which have been reviewed systematically and recently found to be inversely linked to diabetes development [24]. Antioxidants in fruits and vegetables have been hypothesized to protect against diabetes, suspending oxidative stress accumulation, which inflicts damage on the pancreatic beta-cell's dynamic for insulin production and secretion [9]. Yet, supplementation with β-carotene and vitamins C and E has reported null associations with the risk of developing diabetes in women at high risk of CVD [25]. The interplay of a complex mixture of fruits and vegetables found in whole fruits and vegetables may be the key to healthy nutritional behavior [26]. Bread, pasta, and rusk are also present in this pattern. It has been suggested that grain-oriented dietary fibers may act favorably against diabetes development because they cause delayed gastric emptying, which results in a decelerated glucose absorption, thus reducing the insulin level that the pancreatic beta-cells have to provide [27]. The bran of cereals is also very high in magnesium, which has been much studied in relation to diabetes. Decreased intracellular enzymatic activity due to magnesium deficiency promotes insulin resistance [28]. Legumes are excellent sources of magnesium and dietary fibers. Finally, the vegetarian-based pattern is poor in total and saturated fat. Consumption of dietary patterns high in total and saturated fat is directly and indirectly (fostering overweight) linked to impaired insulin resistance [29]. In summary, it is believed that single foods may have antidiabetic properties, but the antidiabetic potential of a healthful dietary pattern is postulated to be higher than the combined effects of single nutritional components.
We have applied statistical analysis of the data so as to produce as robust estimates as possible. Because the exact time of diabetes onset was unknown in all cases (only the date of diabetes diagnosis was known), the hazard ratios were estimated through odds ratios, which may have overestimated the true effect. However, for low-frequency diseases, it is suggested that odds ratio is an accurate estimate (converging) of the relative risk. Furthermore, an underestimation of diabetes incidence was possible considering that individuals with CVD were excluded at baseline assessment. Another limitation was that a misclassification of diabetes status was possible for patients interviewed by phone. Also, the baseline nutritional evaluation was performed once only, thus not reflecting possible seasonal variation, and not allowing reproduction of the information collected. However, the food frequency questionnaire used has been found to be reproducible and reliable, while the sampling was performed over a year, and therefore, on average, nutritional habits from all seasons were included.
Stratification of the sample by age group may have led to decreased power and robustness in estimations of the effect sizes. However, the stratification was deemed necessary because of the significant interaction that existed. The lost-to follow-up rate was medium (about 15%), and mainly attributed to the wrong or missing contact information at baseline; this may have influenced our findings. However, it should be mentioned that only slight differences in the baseline characteristics were observed between those who participated in the follow-up and those who were lost. Finally, the fact that some participants might have changed their dietary habits during the long follow-up of 10 years, without timely information updates, as well as residual confounding due to unmeasured factors, are common limitation of this type of study.
5. Conclusions
The present work confirmed the protective effect of a pattern high in fruits, vegetables, legumes, and grains against diabetes development. The novelty is that the healthful dietary pattern included plant-based foods only, no fish, dairy, or poultry, and that the antidiabetic effect was documented only among middle-aged participants who are at a critical age for diabetes development. Since diet is a lifestyle factor that people can learn to control, this work carries a significant public health message, underlining the role of adherence to a plant-based pattern not only for diabetes, but also for primary prevention of a wide range of cardio-metabolic diseases.
Funding: The ATTICA study was funded by research grants from the Hellenic Cardiology Society (HCS/2002), and the Hellenic Atherosclerosis Society (HAS/2004/2015). Demosthenes Panagiotakos and Ekavi Georgousopoulou received research grants from Coca-Cola SA (10.9.2013).
Authorship: EK performed the literature search and statistical analysis, and wrote the paper. EG, AG, AC, MC, CC, and DT carried out data collection and critically reviewed the paper. CS and CP designed the study. DBP was guarantor of the work; he designed the study, supervised the data analysis, and critically reviewed the paper.
Acknowledgments:
The authors would like to thank the following investigators from the ATTICA Study Group: Yannis Skoumas, Natassa Katinioti, Labros Papadimitriou, Constantina Masoura, Spiros Vellas, Yannis Lentzas, Manolis Kambaxis, Konstadina Palliou, Vassiliki Metaxa, Agathi Ntzouvani, Dimitris Mpougatsas, Nikolaos Skourlis, Christina Papanikolaou, Georgia-Maria Kouli, Aimilia Christou, Adella Zana, Maria Ntertimani, Aikaterini Kalogeropoulou, Evangelia Pitaraki, Alexandros Laskaris, Mihail Hatzigeorgiou, and Athanasios Grekas for their assistance in the initial physical examination and follow-up evaluation. We are also grateful to Efi Tsetsekou for her assistance in psychological evaluation, and to the laboratory team, including Carmen Vassiliadou and George Dedoussis (genetic analysis), Marina Toutouza-Giotsa, Constadina Tselika, and Sia Poulopoulou (biochemical analysis), and Maria Toutouza (database management).
References
- Mathers CD, Loncar D. Projections of global mortality and burden of disease from 2002 to 2030. Plos Med 2006. 3(11):e442. [DOD] [CrossRef]
- Mendis S, Davis S, Norrving B. Organizational update: the world health organization global status report on noncommunicable diseases 2014; one more landmark step in the combat against stroke and vascular disease. Stroke 2015. 46(5):e121-e122. [DOD] [CrossRef]
- Fox CS, Coady S, Sorlie PD, D'Agostino RB, Pencina MJ Sr, Vasan RS, Meigs JB, Levy D, Savage PJ. Increasing cardiovascular disease burden due to diabetes mellitus: the Framingham Heart Study. Circulation 2007. 115(12):1544-1550. [DOD] [CrossRef]
- Mayor S. International Diabetes Federation consensus on prevention of type 2 diabetes. Int J Clin Pract 2007. 61(10):1773-1775. [DOD] [CrossRef]
- Khazrai YM, Defeudis G, Pozzilli P. Effect of diet on type 2 diabetes mellitus: a review. Diabetes Metab Res Rev 2014. 30(Suppl 1):24-33. [DOD] [CrossRef]
- Salas-Salvado J. The role of diet in the prevention of type 2 diabetes. Nutr Metab Cardiovasc Dis 2011. 21(Suppl 2):B32-B48. [DOD] [CrossRef]
- Panagiotakos DB, Pitsavos C, Stefanadis C. Inclusion of dietary evaluation in cardiovascular disease risk prediction models increases accuracy and reduces bias of the estimations. Risk Anal 2009. 29(2):176-186. [DOD] [CrossRef]
- Brunner EJ, Mosdol A, Witte DR, Martikainen P, Stafford M, Shipley MJ, Marmot MG. Dietary patterns and 15-y risks of major coronary events, diabetes, and mortality. Am J Clin Nutr 2008. 87(5):1414-1421. [DOD]
- Martinez-Gonzalez MA, de la Fuente-Arrillaga C, Nunez-Cordoba JM, Basterra Gortari FJ, Beunza JJ, Vazquez Z, Benito S, Tortosa A, Bes-Rastrollo M. Adherence to Mediterranean diet and risk of developing diabetes: prospective cohort study. BMJ 2008. 336(7657):1348-1351. [DOD] [CrossRef]
- Koloverou E, Panagiotakos DB, Pitsavos C, Chrysohoou C, Georgousopoulou EN, Grekas A, Christou A, Chatzigeorgiou M, Skoumas I, Tousoulis D, et al. Adherence to Mediterranean diet and 10-year incidence (2012-2014) of diabetes; the mediating effect of inflammatory and oxidative stress biomarkers: results from ATTICA cohort study. Diabetes Metab Res Rev 2015. 32(1):73-81. [DOD] [CrossRef]
- Salas-Salvado J, Martinez-Gonzalez MA, Bullo M, Ros E. Reduction in the incidence of type 2 diabetes with the Mediterranean diet: results of the PREDIMED-Reus nutrition intervention randomized trial. Diabetes Care 2011. 34(1):14-19. [DOD] [CrossRef]
- Willett WC, Sacks F, Trichopoulou A, Drescher G, Ferro-Luzzi A, Helsing E, Trichopoulos D. Mediterranean diet pyramid: a cultural model for healthy eating. Am J Clin Nutr 1995. 61(6 Suppl):1402S-1406S. [DOD]
- van Dam RM, Rimm EB, Willett WC, Stampfer MJ, Hu FB. Dietary patterns and risk for type 2 diabetes mellitus in U.S. men. Ann Intern Med 2002. 136(3):201-209. [DOD] [CrossRef]
- Hodge AM, English DR, O'Dea K, Giles GG. Dietary patterns and diabetes incidence in the Melbourne Collaborative Cohort Study. Am J Epidemiol 2007. 165(6):603-610. [DOD] [CrossRef]
- Maghsoudi Z, Ghiasvand R, Salehi-Abargouei A. Empirically derived dietary patterns and incident type 2 diabetes mellitus: a systematic review and meta-analysis on prospective observational studies. Public Health Nutr 2016. 19(2):230-241. [DOD] [CrossRef]
- Zaroudi M, Yazdani Charati J, Mehrabi S, Ghorbani, E, Norouzkhani J, Shirashiani H, Nikzad B, Seiedpour M, Izadi M, Mirzaei M, et al. Dietary Patterns Are Associated with Risk of Diabetes Type 2: A Population-Based Case-Control Study. Arch Iran Med 2016. 19(3):166-172. [DOD]
- Koloverou E, Panagiotakos DB, Pitsavos C, Chrysohoou C, Georgousopoulou EN, Pitaraki E, Metaxa V, Stefanadis C. 10-year incidence of diabetes and associated risk factors in Greece: the ATTICA study (2002-2012). Rev Diabet Stud 2014. 11(2):181-189. [DOD] [CrossRef]
- Pitsavos C, Panagiotakos DB, Chrysohoou C, Stefanadis C. Epidemiology of cardiovascular risk factors in Greece: aims, design and baseline characteristics of the ATTICA study. BMC Public Health 2003. 3:3-32. [DOD] [CrossRef]
- Katsouyanni K, Rimm EB, Gnardellis C, Trichopoulos D, Polychronopoulos E, Trichopoulou A. Reproducibility and relative validity of an extensive semi-quantitative food frequency questionnaire using dietary records and biochemical markers among Greek schoolteachers. Int J Epidemiol 1997. 26(Suppl 1):S1181-S1127. [DOD]
- Papathanasiou G, Georgoudis G, Papandreou M, Spyropoulos P, Georgakopoulos D, Kalfakakou V, Evangelou A. Reliability measures of the short International Physical Activity Questionnaire (IPAQ) in Greek young adults. Hellenic J Cardiol 2009. 50(4):283-294. [DOD]
- American Diabetes Association. Report of the Expert Committee on the Diagnosis and Classification of Diabetes Mellitus. Diabetes Care 1997. 20(7): 1183-1197. [DOD] [CrossRef]
- Panagiotakos D. A-priori versus a-posterior methods in dietary pattern analysis: a review in nutrition epidemiology. Nutrition Bulletin 2008. 33:311-315. [DOD] [CrossRef]
- Montonen J, Knekt P, Harkanen T, Jarvinen R, Heliovaara M, Aromaa A, Reunanen A. Dietary patterns and the incidence of type 2 diabetes. Am J Epidemiol 2005. 161(3):219-227. [DOD] [CrossRef]
- Cooper AJ, Forouhi NG, Ye Z, Buijsse B, Arriola L, Balkau B, Barricarte A, Beulens JW, Boeing H, Buchner FL, et al. Fruit and vegetable intake and type 2 diabetes: EPIC-InterAct prospective study and meta-analysis. Eur J Clin Nutr 2012. 66(10):1082-1092. [DOD] [CrossRef]
- Song Y, Cook NR, Albert CM, Van Denburgh M, Manson JE. Effects of vitamins C and E and beta-carotene on the risk of type 2 diabetes in women at high risk of cardiovascular disease: a randomized controlled trial. Am J Clin Nutr 2009. 90(2):429-437. [DOD] [CrossRef]
- Liu RH. Health benefits of fruit and vegetables are from additive and synergistic combinations of phytochemicals. Am J Clin Nutr 2003. 78(3 Suppl):517S-520S. [DOD]
- Liese AD, Schulz M, Fang F, Wolever TM, D'Agostino RB Jr. Sparks KC, Mayer-Davis EJ. Dietary glycemic index and glycemic load, carbohydrate and fiber intake, and measures of insulin sensitivity, secretion, and adiposity in the Insulin Resistance Atherosclerosis Study. Diabetes Care 2005. 28(12):2832-2838. [DOD] [CrossRef]
- Barbagallo M, Dominguez LJ, Galioto A, Ferlisi A, Cani C, Malfa L, Pineo A, Busardo A, Paolisso G. Role of magnesium in insulin action, diabetes and cardio-metabolic syndrome X. Mol Aspects Med 2003. 24(1-3):39-52. [DOD] [CrossRef]
- Riccardi G, Giacco R, Rivellese AA. Dietary fat, insulin sensitivity and the metabolic syndrome. Clin Nutr 2004. 23(4):447-456. [DOD] [CrossRef]
|