Chapter II. Prevention and Treatment
Rev Diabet Stud,
2015,
12(3-4):351-362 |
DOI 10.1900/RDS.2015.12.351 |
Type 2 Diabetes Prevention: Implications of Hemoglobin A1c Genetics
Aaron Leong1,2, James B. Meigs1,2
1Massachusetts General Hospital, General Medicine Division, Boston, MA, USA
2Harvard Medical School, Boston, MA, USA
Address correspondence to: Aaron Leong, e-mail: asleong@mgh.harvard.edu
Manuscript submitted May 3, 2015; resubmitted June 29, 2015; accepted July 22, 2015.
Keywords: type 2 diabetes, HbA1c, fasting glucose, GWAS, glycemic pathway, glycemic trait, index SNP
Abstract
Hemoglobin A1c (HbA1c) is a biomarker used for population-level screening of type 2 diabetes (T2D) and risk stratification. Large-scale, genome-wide association studies have identified multiple genomic loci influencing HbA1c. We discuss the challenges of classifying these genomic loci as influencing HbA1c through glycemic or nonglycemic pathways, based on their probable biology and pleiotropic associations with erythrocyte traits. We show that putative nonglycemic genetic variants have a measurable, albeit small, impact on the classification of T2D status by HbA1c in white and Asian populations. Accounting for their effect on HbA1c may be relevant when screening populations with higher frequencies of nonglycemic HbA1c-altering alleles. As carriers of such HbA1c-altering alleles have HbA1c levels that may not accurately reflect overall glycemia, we describe how accounting for genotype may improve the performance of HbA1c in T2D prediction models and risk stratification, allowing for lifestyle intervention strategies to be directed towards those who are truly at elevated risk for developing T2D. In a Mendelian randomization framework, genetic variants can be used as instrumental variables to estimate causal relationships between HbA1c and T2D-related complications. This approach may help to support or refute HbA1c as an appropriate biomarker for long-term health outcomes in the general population.
Abbreviations: ABCB11 - ATP-binding cassette, subfamily B, member 11; 2hrG - 2-hour glucose; AGEN - Asian Genetics Epidemiology Network; DCCT - Diabetes Control and Complications Trial; DIAGRAM - Diabetes Genetics Replications and Meta-Analysis; ENCODE - Encyclopedia of DNA Elements; FG - fasting glucose; FEV1 - forced expiratory volume in 1 second; FN3K - fructosamine-3-kinase; GCK - glucokinase; GWAS - genome-wide association study; GRASP - Genome-Wide Repository of Associations Between SNPs and Phenotypes; HbA1c - hemoglobin A1c; HFE - human hemochromatosis protein; LD - linkage disequilibrium; MCHC - mean corpuscular hemoglobin concentration; MR - Mendelian randomization; MAGIC - Meta-Analysis of Glycemic and Insulin-Related Traits Consortium; NGSP - National Glycohemoglobin Standardization Program; PI - proinsulin; RBC - red blood cell; SLC30A8 - solute carrier family 30 (zinc transporter), member 8; SNP - single nucleotide polymorphism; T2D - type 2 diabetes; TCF7L2 - transcription factor 7 like 2; UKPDS - United Kingdom Prospective Diabetes Study; UCSC - University of California, Santa Cruz
1. Introduction
In the era of precision medicine, we witness a growing emphasis on personalized healthcare. While precision medicine has often been associated with targeted pharmacologic therapies, its overarching goal extends to other aspects of healthcare, e.g., customized prevention strategies, appropriate diagnostic testing, and the use of biomarkers for disease prediction and health screening [1, 2]. Hemoglobin A1c (HbA1c) is a biomarker that has been used for type 2 diabetes (T2D) diagnosis and prediction [3]. The HbA1c test measures the proportion of glycated hemoglobin in the blood, an irreversible chemical modification of the hemoglobin molecule by blood glucose, which reflects ambient glycemia averaged over the preceding 2-3 months [4], the average life span of a red blood cell (RBC). HbA1c levels are in part genetically determined. Studies on nondiabetic twins reported heritability estimates for HbA1c levels of around 57-75%, whereas the heritability of fasting glucose (FG) was less, 38-66% [5, 6]. While previous cross-sectional examinations have shown generally good agreement between HbA1c and FG levels for diagnosing T2D in varying populations, some ethnic differences exist [7-10]. It also remains unclear to what extent the genetic factors that influence HbA1c and FG are shared [5, 6], and whether these shared genetic factors are important in T2D prediction.
HbA1c is the only test for T2D that is not directly a measurement of blood glucose [3]. While there is strong evidence supporting the use of HbA1c to identify individuals with early dysglycemia, nonglycemic factors can influence HbA1c variability in populations. These include hematologic conditions that affect RBC turnover or shorten RBC intravascular lifespan (e.g. hereditary hemolytic anemia and blood loss), and falsely lower HbA1c levels [11], iron deficiency anemia, and iron deficiency that have been associated with higher HbA1c readings [12, 13]. Chemical modifications of hemoglobin (e.g., carbamylated hemoglobin in renal failure), hemoglobin variants (e.g. HbS, HbC, HbD, HbE), and elevated fetal hemoglobin can interfere with some laboratory assay methods and profoundly affect measurement accuracy [14, 15]. Notably, some of these nonglycemic factors affecting measured HbA1c levels are genetically determined.
This review has two sequential parts. Firstly, we summarize the main genetic discoveries from pivotal genome-wide association studies (GWAS) on HbA1c in the last 8 years, and discuss mechanisms by which these GWAS genomic loci may influence HbA1c levels. Secondly, we highlight the potential public health and clinical implications of HbA1c genetics with respect to T2D population screening and prediction.
2. Discovery of HbA1c-related genomic loci
2.1 HbA1c discovery GWAS
To date, discovery GWAS have identified 17 HbA1c-related genomic loci. In 2008, Pare and colleagues conducted a GWAS on 14,618 nondiabetic participants of European ancestry in the Women's Genome Health Study [16]. They reported that HbA1c was associated with genetic variation at four genomic loci (GCK, rs730497, p = 2.8 x 10-12; SLC30A8, rs13266634, p = 9.8 x10-8, G6PC2/ ABCB11, rs1402837, p = 6.8 x 10-10; HK, rs7072268; p = 6.4 x10-9). In 2010, Soranzo and colleagues conducted a larger meta-analysis of GWAS on 46,368 nondiabetic adults of European ancestry in the Meta-analysis of Glycemic and Insulin-related traits Consortium (MAGIC). They reported 10 genomic loci associated with HbA1c, six of which were novel, FN3K (rs1046896, p = 1.6 x 10-26), HFE (rs1800562, p = 2.6 x 10-20), TMPRSS6 (rs855791, p = 2.7 x 10-14), ANK1 (rs4737009, p = 6.1 x10-12), ATP11A/TUBGCP3 (rs7998202, p = 5.2 x 10-9), SPTA1 (rs2779116, p =2.8 x 10-9), and four loci that had been reported to be associated with HbA1c (HK1, GCK, G6PC2/ABCB11, and MTNR1B) [17].
This meta-analysis of GWAS was followed closely by a smaller GWAS (n = 1,782) conducted by Franklin and colleagues. The authors reported a variant in an intron of TCF7L2 associated with HbA1c at subthreshold GW significance (rs7903146, p = 1.48 x 10-7) [18]. In 2012, Ryu and Lee conducted a GWAS in an Asian population (n = 8,057) within the Korean Association Study, and identified novel HbA1c-associated genetic variants in CDKAL1; the strongest signal being an intronic variant, rs7747752 (p = 1.0 x 10-11) [19]. In 2014, Chen and colleagues published the second-largest meta-analysis of HbA1c GWAS on 21,026 individuals from East Asian cohorts in the consortium of the Asian Genetics Epidemiology Network (AGEN). This study reported nine variants at genome-wide significance, four of which were novel (TMEN79, rs6684514, p = 1.3 x 10-23; HB21L/ MYB, rs9399137, p = 8.5 x 10-15, MYO9B, rs11667918, p = 9.0 x 10-12, and CYBA, rs9933309, p = 1.1 x 10-28), one in an intron of CDKAL1, rs7772603, a locus previous implicated in the Korean study, and four loci (G6PC2/ABCB11, GCK, ANK1, and FN3K) which were previously identified by MAGIC [20]. This result demonstrated that HbA1c variants carried by Europeans are generally transferable to East Asians. It is anticipated that larger trans-ethnic meta-analyses of GWAS may be required to boost the statistical power for discovering novel HbA1c-related loci, and detecting genetic variants at lower frequencies.
2.2 Glycemic and nonglycemic pathways: genetic architecture of HbA1c in relation to that of T2D and related traits
Using a combination of cross-trait association analyses, mediation analyses, and known biological function of nearby genes, previous GWAS have attempted to classify 17 discovered HbA1c-related variants as possibly "glycemic" or "nonglycemic" [17, 20]. Figure 1 depicts overlapping sets of HbA1c-related loci with T2D, FG, proinsulin (PI), and 2-hr glucose (2hrG) GWAS loci; six were considered glycemic as they were associated at genome-wide significance with either FG or T2D, or both, three of which were additionally associated with fasting PI or 2hrG. Notably, none of the HbA1c-related loci were associated with fasting insulin (FI) at genome-wide significance [21-23]. Index single nucleotide polymorphisms (SNPs) within the six glycemic loci were as follows:
1. rs7903146: an intronic variant of TCF7L2, a gene encoding a transcription factor involved in the Wnt signaling pathway and the proliferation of pancreatic beta-cells [24].
2. rs13266634: a missense mutation in SLC30A8, encoding a zinc transporter associated with beta-cell function [25, 26] which led to the discovery of rare protein-truncating SLC30A8 variants, conferring T2D protection [27].
3. rs1387153: a variant 29 kb from the 5' end of MTNR1B, encoding the receptor for melatonin, the principle hormone secreted by the pineal gland that may coordinate circadian patterns and insulin secretion [28, 29].
4. rs1799884: a variant 45 bp from the 5' end of GCK, encoding glucokinase, a hexokinase that phosphorylates glucose, the initial step in glucose metabolism pathways primarily in the liver and pancreas [30].
5. rs7772603: an intronic SNP in CDKAL1, encoding a transfer ribonucleic acid (tRNA) modification enzyme that may influence insulin secretion [31].
6. rs552976: an intronic variant in ABCB11, encoding an ABC transporter, bile salt export pump, involved in hepatic bile production [32]. This particular SNP is in close linkage disequilibrium (LD ≥ 0.8) with other SNPs in the intergenic region near G6PC2, which encodes a key enzyme in the terminal step of gluconeogenic and glycogenolytic pathways [33].
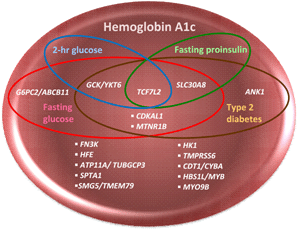 |
 |
Figure 1. Venn diagram of genomic loci associated with HbA1c, fasting glucose, fasting proinsiulin, 2-hour glucose, and type 2 diabetes detected by GWAS. This Venn diagram depicts overlapping sets of HbA1c-related genomic loci which are also associated with type 2 diabetes (T2D, Mahajan et al. 2014, DIAbetes Genetics Replication and Meta-analysis (DIAGRAM) consortium), fasting glucose (FG), fasting insulin (FI), 2-hour glucose (2hrG, Scott et al., 2012, Meta-Analysis of Glucose and Insulin related traits Consortium (MAGIC)), and fasting proinsulin (PI, Strawbridge et al., 2011, MAGIC) in GWAS [21-23]. PI, a precursor of insulin in secretory granules of the pancreatic beta-cell, has minimal insulin activity until it has been processed to insulin. Elevated circulating levels of PI are associated with impaired beta-cell function, decreased insulin processing or secretion, or "beta-cell stress" resulting from insulin resistance [87]. PI, similarly to HbA1c, FG, 2hrG, and FI, is a predictor of future T2D [88]. Six HbA1c GWAS loci are glycemic: one (G6PC2/ABCB11) is associated with FG only, and five are associated with both T2D and FG GWAS loci, with three of these five (TCF7L2, GCK/YKT6, and SCL30A8) also associating with either 2hrG or PI. The HbA1c index SNP in ANK1 was considered "nonglycemic" when it was first discovered, although a T2D index SNP within ANK1 has been found in later GWAS. None of the HbA1c GWAS loci overlap with FI. |
|
These genetic variants implicate diverse biochemical pathways underlying T2D risk, glucose regulation, and subsequent glycemia quantified by elevated HbA1c values.
The remaining 11 HbA1c-related genomic loci have been classified as possibly "nonglycemic". Seven (FN3K, HFE, TMPRSS6, ANK1, SPTA1, ATP11A, and HK1) were first reported in European populations [16, 17], and the remaining four (TMEN79, HB21L/MYB, MYO9B, and CYBA) were reported in Asian populations [20]. These genomic loci were either not strongly associated with FG, remained associated with HbA1c even after adjustment for FG in mediation analyses, or were strongly associated with red blood cell traits (e.g. hemoglobin levels, mean corpuscular volume, and mean corpuscular hemoglobin concentration) in cross-trait look-ups [17, 20].
While some genomic loci were easily classified as possibly "glycemic" or "nonglycemic", it was more challenging in the case of others to assign a potential mechanistic relationship to HbA1c levels. For instance, HK1, encoding hexokinase, was considered "glycemic" when it was first reported by Pare et al. in 2008 (rs7072268, intron in HK1, p = 6.4 x 10-9) [16]. Hexokinase is an enzyme that phosphorylates glucose to produce glucose-6-phosphate, the initial step in glucose metabolism. While hexokinase-1 is ubiquitously expressed, its presence is important in RBCs as they depend exclusively on glycolysis for ATP generation and energy production. Several other lines of evidence indicate that HK1 may influence RBC biology:
1. Mutations in HK1 are associated with hexokinase deficiency and hereditary nonspherocytic hemolytic anemia [34, 35].
2. In Soranzo et al., the association between the lead SNP in HK1 (rs16926246-C, p = 3.1 x 10-54) and HbA1c did not attenuate substantially with adjustment for FG (unadjusted β = 0.073 (SE 0.007) % points, p = 4.8 x 10-26 vs. FG-adjusted β = 0.069 (0.006), p = 6.4 x 10-30) [17].
3. HK1 was associated with hemoglobin levels in population-based cohorts that contributed to this meta-analysis of GWAS (p = 1.1 x 10-3, n = 7,534) [36]. It is possible that variations in HK1 may affect HbA1c via pleiotropic mechanisms, involving both glucose metabolism and RBC biology, e.g., impaired intra-cellular glucose metabolism that impact RBC survival.
Another genomic locus that did not classify cleanly is ANK1, a gene encoding ankyrin that connects integral membrane proteins to underlying spectrin-actin cytoskeleton [37]. Mutations in erythrocytic ankyrin 1 result in ankyrin-deficient, hereditary spherocytosis [38]. In 2010, Soranzo et al. identified two independent HbA1c GWAS signals (r2 = 0.009) residing in introns of ANK1, rs6474359-T (risk allele frequency = 0.97, β = 0.06 (0.04-0.08), p = 1.2 x 10-8) and rs4737009-A (risk allele frequency = 0.24, β = 0.03 (0.02-0.04), p = 6.1 x 10-12) [17]. Mediation analyses indicated that ANK1 likely influenced HbA1c independently of FG. Given the known biological function of ANK1, the authors concluded that this genomic locus likely influenced HbA1c levels through mechanisms involving RBC biology and not glucose regulation. Using the Genome-Wide Repository of Associations Between SNPs and Phenotypes (GRASP) database, SNPs in ANK1 was also found to be associated with mean corpuscular hemoglobin concentration (MCHC; rs4737009; p = 4.9 x 10-11; n =135,367) in a large meta-analysis of GWAS on RBC phenotypes [39].
In 2014, a trans-ethnic meta-analysis of GWAS in T2D by the Diabetes Genetics Replications and Meta-Analysis (DIAGRAM) consortium reported a T2D lead SNP, rs516946-C (risk allele frequency = 0.77, OR 1.10 (1.06-1.15), p = 2 x 10-7), residing in the same ANK1 locus, specifically in an intron closer to the protein-coding exon. This SNP may be regarded as a third independent signal (r2 = 0.004). These findings challenge the notion that ANK1 influences HbA1c via RBC biology only. Incidentally, another independent SNP, rs7006290 (r2 = 0.05), in the ANK1 locus has been associated with pulmonary function decline (forced expiratory volume in 1 second (FEV1) decline) in asthmatics (p = 5.2 x 10-6, n = 4,118). Indeed, variations in ANK1 may have pleiotropic effects on different diseases and phenotypic traits. Figure 2 features a UCSC (University of California, Santa Cruz) browser snapshot of the ANK1 locus, chr8:41,460,000-41,760,000 (300 kb), containing GWAS SNPs for T2D, HbA1c, FEV1 decline, and MCHC. The HbA1c-related SNP, rs4737009, falls on a putative enhancer (H3KMe1 histone mark), a DNase 1 hypersensitivity area, and 12 transcription factor binding sites derived from ChIP-seq experiments, 3 of which have canonical motifs for the corresponding factor. Detailed annotation of the genomic region in close proximity to the HbA1c index SNP illuminated functional elements that potentially regulate gene expression. Laboratory experiments designed to test whether genetic variation in this locus affects transcript expression in various tissue types may offer deeper insight into the pleiotropic effects exerted by ANK1.
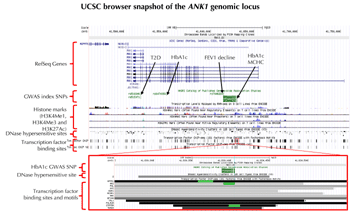 |
 |
Figure 2. Genetic variants in the ANK1 genomic locus and their associated phenotypes. This figure is a UCSC browser snapshot of the ANK1 genomic locus, chr8:41,460,000-41,760,000 (300 kb), illustrating the relative positions of GWAS index SNPs for type 2 diabetes (T2D), hemoglobin A1c (HbA1c), forced expiratory volume in 1 second (FEV1) decline, and mean corpuscular hemoglobin concentration (MCHC) residing in introns of ANK1. The figure also shows Encyclopedia of DNA Elements (ENCODE) functional elements located near the HbA1c index SNP, rs4737009. These include an H3KMe1 histone mark, which is suggestive of a putative enhancer, and a DNase 1 hypersensitivity site, indicating a DNase-sensitive regulatory region. The zoomed-in plot shows that this HbA1c index SNP falls within 12 transcription factor binding sites (TFBS) derived from ChIP-seq experiments. These are demarcated by gray boxes. Three of these TFBS have canonical motifs for the corresponding factor (green highlight), further suggesting that the region has regulatory function impacting gene expression. |
|
HbA1c genetics provide an additional line of evidence for the genetic determinants of dysglycemia, allowing us to map out better the genetic architecture of T2D and related traits. Risk alleles may be present in higher frequencies in certain populations [40-43], which may partly explain some of the ethnic disparities in T2D risk and population distribution of HbA1c. Prioritizing genomic loci that are associated with multiple T2D-related traits for targeted sequencing and detailed laboratory experimentation with a focus on tissue-specific transcript expression may be a fruitful strategy for identifying causal variants. Findings may suggest mechanisms that mediate genetic effects in T2D-susceptible persons, potentially unveiling novel therapeutic targets for personalized medicine. While glycemic HbA1c variants may be particularly relevant for revealing biological pathways related to T2D susceptibility, nonglycemic HbA1c variants contribute to the inaccuracy of HbA1c as a population-level screening tool for T2D detection and risk stratification.
3. Implications for T2D diagnosis and population screening
In 2010, the American Diabetes Association (ADA) recommended the use of HbA1c as one of three biochemical criteria for diagnosing clinical diabetes. Since then, 6.5% has been widely adopted as the HbA1c threshold for clinical diagnosis of T2D [3]. Putative nonglycemic HbA1c genetic variants may result in the misclassification of individuals around this HbA1c threshold for T2D diagnosis. Table 1 shows two net reclassification analyses to estimate the population-level impact of putative nonglycemic HbA1c loci on screening for undiagnosed T2D; the first analysis was by Soranzo et al. in European samples, the second by Chen et al. in Asian samples [17, 20].
Putative nonglycemic HbA1c-associated SNPs affect erythrocyte turnover or abnormal glycation of hemoglobin, which may contribute to elevated or reduced HbA1c relative to glycemia. To determine the impact of these SNPs on T2D classification, Soronzo and colleagues calculated the net reclassification around the 6.5% threshold for T2D diagnosis attributable to the effects of seven putative nonglycemic HbA1c loci (FN3K, HFE, TMPRSS6, ANK1, SPTA1, ATP11A, and HK1) compared to undiagnosed T2D, defined by FG ≥ 7 mmol/l, in 10,110 participants from the Framingham Heart Study and the Atherosclerosis Risk in Communities Study [17]. The investigators first excluded individuals who either had a physician-diagnosed T2D or who were taking anti-diabetic medication. To identify the proportion of individuals whose T2D diagnosis by measured HbA1c ≥ 6.5% were reclassified after adjusting for the contribution of the seven nonglycemic genetic variants, the authors calculated a SNP-adjusted HbA1c by increasing or decreasing the measured HbA1c by the difference between the number of HbA1c-raising alleles (0, 1, or 2) and 2 times the allelic frequency in the sample, multiplied by the effect size of each nonglycemic SNP. Subsequently, they constructed two-by-two tables representing the proportion of individuals whose T2D diagnoses were discordant by measured HbA1c and SNP-adjusted HbA1c (Table 1). Of 593 individuals (5.9% of total sample) with undiagnosed T2D (FG ≥ 7 mmol/l), 234 (39.5%) had measured HbA1c ≥ 6.5%, and 222 (37.4%) had a SNP-adjusted HbA1c ≥ 6.5%. This means that 2% of those with FG ≥ 7 mmol/l had a measured HbA1c ≥ 6.5%, which was adjusted to < 6.5% after accounting for the contribution of the seven putative non-glycemic SNPs (Figure 3). While FG was used as the reference standard in this study, we acknowledge that FG has greater biological variability than HbA1c, and so it remains unclear whether a single measurement of FG is a better screening test than measured HbA1c or SNP-adjusted HbA1c.
Table
1.
Reclassification table of measured HbA1c and nonglycemic HbA1c SNP-adjusted HbA1c to estimate the population-level impact of nonglycemic HbA1c SNPs on T2D screening in European and Asian cohorts |
|
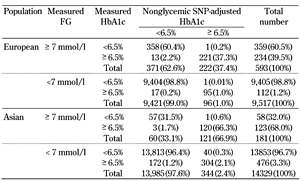 |
 |
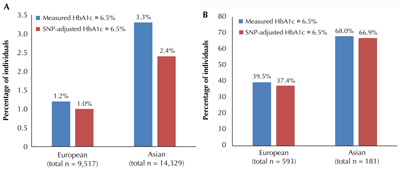 |
 |
Figure 3. Reclassification of non-T2D (A) and undiagnosed T2D individuals (B) as T2D based on measured HbA1c and HbA1c adjusted for nonglycemic SNPs around the HbA1c threshold of 6.5% in European and Asian cohorts. A: Reclassification of non-T2D individuals, defined by FG < 7mmol/l, around the HbA1c threshold of 6.5%, attributable to nonglycemic SNPs. Reclassification was more evident in Asians, where 3.3% of non-T2D individuals were classified as T2D based on measured HbA1c, but only 2.4% after accounting for nonglycemic SNPs. The equivalent comparison in Europeans was minimal -1.2% vs. 1.0%. B: Reclassification of undiagnosed T2D individuals, defined by FG ≥ 7 mmol/l without treatment, around the HbA1c threshold of 6.5% attributable to nonglycemic SNPs. Here, reclassification was subtle for both Asian and European cohorts. The diagrams were adapted from Soranzo et al. [17] (A) and Chen et al. [20] (B). |
|
Chen and colleagues addressed the population impact of six putative nonglycemic HbA1c SNPs (TMEN79, HB21L/MYB, MYO9B, CYBA, ANK1, and FN3K) in cohorts of Asian ancestry (n = 15,150) using a similar reclassification analysis. In this study, the proportion of undiagnosed T2D based on FG ≥ 7mmol/l was smaller than in the European study (1.2% (n = 181) in Asians vs. 5.9% in Europeans). About two in three individuals with undiagnosed T2D (n = 123 (68.0%)) in this Asian study had measured HbA1c ≥ 6.5%. Adjusting for the putative nonglycemic loci did not result in much net reclassification. However, there was marked reclassification among the 14,329 individuals without T2D (i.e. FG < 7mmol/l), with 476 (3.3%) nondiabetic individuals classified as having T2D according to measured HbA1c ≥ 6.5%, but only 344 (2.4%) after adjusting for putative nonglycemic HbA1c SNPs. The equivalent comparison in Europeans was 1.2% vs. 1.0%. Thus, reclassification of non-T2D individuals in Asian populations was much larger than in European populations, implying that the population impact of putative nonglycemic genetic factors on reclassification around the HbA1c threshold of 6.5% may differ by ancestry. Similar analyses are needed in populations of non-European ancestry, such as Africans, where selective pressure by infectious diseases on RBC lifespan, polymorphism, or hemoglobin variants [44-46] may have leveraged HbA1c-influencing, RBC-related alleles to reach greater frequency.
Aggregating data from populations of different ancestry in large-sample, trans-ethnic meta-analyses of GWAS will provide power to detect additional HbA1c-related genomic loci, allowing a deeper examination of the transferability of HbA1c-related genetic variants to different ethnicities [21]. It is noteworthy that an investigation in African Americans has shown that the relative contribution of demographic and metabolic factors to measured HbA1c is considerably larger than the contribution of genetic ancestry. Adjusting for genetic ancestry, after accounting for fasting glucose, has minimal impact on T2D classification by HbA1c, suggesting that ethnicity-specific, diagnostic thresholds are not warranted for population screening [47]. Nevertheless, in populations with a higher prevalence of nonglycemic, HbA1c-altering alleles, employing genotype-specific, diagnostic thresholds, or calibrating measured HbA1c according to an individual's genotype profile to account for the effect of nonglycemic, HbA1c-altering alleles, may improve HbA1c's ability to detect undiagnosed T2D cases. Alternatively, glucose measurements, and not HbA1c, can be used to screen individuals with many nonglycemic HbA1c-related variants for T2D, similar to the recommended procedure for individuals with renal failure (carbamylation of hemoglobin), known hemoglobinopathies [14, 15], and other serum protein variants or chemical modifications to hemoglobin that interfere with lab assays. Notably, standardization of lab assay methods across populations is crucial for:
- Estimating ethnic differences in HbA1c levels.
- Making proper inferences about shifts in the distribution of HbA1c over time.
- Estimating trends in the prevalence and control of HbA1c-defined T2D for health care policy and planning [48].
Through GWAS on HbA1c, we may be able to implicate genes that are associated with such lab assay interferences, enabling the development of newer methods to correct such inaccuracies.
4. Implications for T2D risk stratification and prediction
The ADA considers HbA1c levels between 5.7 and 6.4% as "prediabetes", which is supposed to signal an increased risk for future T2D [3]. Indeed, individuals with moderately elevated HbA1c levels have higher incident rates of T2D over time [49-60], supporting the use of HbA1c for risk stratification in the general population. HbA1c is also able to predict future T2D in various non-European populations around the world [9, 10, 51, 53-59, 61]. In the 1990s, the Diabetes Control and Complications Trial (DCCT) [62] and United Kingdom Prospective Diabetes Study (UKPDS) [63] established clear relationships between HbA1c levels and risk of diabetes-related endpoints in both type 1 diabetes and T2D patients. However, technical variation in assay methods initially prevented the optimal use of HbA1c in clinical practice. Therefore, in 1996, the National Glycohemoglobin Standardization Program (NGSP) initiated the harmonization of HbA1c testing to standardize HbA1c results to those used in DCCT and UKPDS [64].
While implementing large-scale measures to promote healthy living in the entire community would be ideal, concentrating T2D prevention efforts on at-risk individuals may be a more cost-effective and feasible strategy. HbA1c thresholds to detect those at-risk for future T2D are, however, not consistent worldwide. The International Expert Committee of the World Health Organization recommended that individuals with HbA1c between 6.0 and 6.5% were at elevated T2D risk and may be considered for T2D prevention strategies [65]. Likewise, the Canadian Diabetes Association chose the cut-point 6.0% to define prediabetes [66], whereas the ADA opted for a lower threshold of 5.7% [3]. As ethnocultural factors, lifestyle/behavioral factors, and other non-genetic factors may modify the predictive value of HbA1c, it is unknown whether these thresholds can or should be uniformly applied in all populations around the world. A cross-sectional study of 4,325 nondiabetic participants in Harbin, China, examined the agreement between HbA1c and oral glucose tolerance for diagnosing T2D and prediabetes [67]. The authors concluded that agreement between HbA1c and OGTT was weaker among obese participants, suggesting that the HbA1c threshold for prediabetes may need to be raised for an obese population.
Cross-sectional examinations in NHANES from 2005 to 2010 indicated that 12.4% of the U.S. population had HbA1c levels between 5.7 and 6.4% [48]. Excluding 9.6% of the U.S. population with T2D or HbA1c ≥ 6.5%, 13.7% (i.e., one in seven nondiabetic U.S. adults) would carry the label "prediabetes". To yield the greatest impact on T2D risk reduction from prevention strategies, interventions should be targeted only at those who are truly at elevated risk for future T2D. Carriers of putative nonglycemic HbA1c-raising alleles with a mildly elevated HbA1c level may fall into this prediabetes category, despite having completely normal glycemia. These individuals would be less likely to benefit from prevention strategies compared to their counterparts with an elevated HbA1c from dysglycemia. Conversely, carriers of putative nonglycemic HbA1c-lowering alleles may be wrongly categorized as "low risk" and "not qualified" for such intervention programs. Population-based cohort studies that test HbA1c as a predictor for T2D, stratified by genotype, race/ethnicity, and other non-genetic factors, may provide better risk estimates in subpopulations.
HbA1c-related genetic variants can be used as genetic instruments in Mendelian randomization (MR) analyses to strengthen causal inferences for the relationship between HbA1c and disease outcomes, particularly T2D-related complications. Studies have shown that HbA1c, even in the nondiabetic range, is associated with incident cardiovascular risk [68, 69]. The association between HbA1c and microvascular complications, retinopathy, and chronic kidney disease is more compelling among individuals with T2D [70, 71]. In fact, the HbA1c cut-point for T2D diagnosis was selected based on epidemiologic evidence from cross-sectional data on different populations showing an abrupt increase in the prevalence of retinopathy above the HbA1c cut-point of 6.5% [3, 66, 71-73]. Nevertheless, some of these observational associations may be partially attributed to confounding or reverse causations. Importantly, randomized controlled trials on intensive vs. conventional therapy in diabetes patients showed that targeting a near-normal HbA1c protected against microvascular disease [74], but not necessarily macrovascular disease [75-80]. Instrumentation in an MR approach can be applied to strengthen or refute a causal relationship between HbA1c and T2D-related complications, supporting the use of HbA1c as a biomarker of disease susceptibility [81].
Applying MR methods for estimating causal associations has limitations and challenges specific to HbA1c. As HbA1c-related common genetic variants explain individually little of the variance in HbA1c, combining multiple variants in a single allelic score for instrumenting HbA1c could reduce the bias from weak instruments and improve the power to detect associations [82-84]. While MR analyses may strengthen causal inference, they do not delineate biological pathways from HbA1c, or hyperglycemia, to outcomes. Accounting for pleiotropy can be challenging as biological functions of some of the HbA1c-related genetic variants are still unknown. Excluding genetic variants that contribute to heterogeneity of effects ensures that estimates from multiple instruments are all similar, but does not specifically evaluate pleiotropy nor take into consideration that instruments may affect different aspects of the intermediate phenotype which in turn have differing effects on disease [85].
A pleiotropy assessment could be performed by excluding HbA1c-related variants that are also correlated with other biomarkers or clinical measures (e.g. body mass index). However, such an approach may be overly conservative as these phenotypes may be intermediates in the causal pathways from hyperglycemia or HbA1c to T2D-related outcomes. Alternatively, carefully selecting genetic variants that are biologically relevant to a specific pathway may produce MR estimates that are more mechanistically interpretable. Notably, when measuring HbA1c, we are agnostic to the underlying glycemic and non-glycemic pathways influencing HbA1c. Therefore, allelic scores, composed of all known HbA1c-related variants that explain more of the variance, may arguably be better for estimating the overall effect of HbA1c on disease risk, even if non-glycemic and other pleiotropic mechanisms partly account for the association. To test the robustness of MR results, sensitivity analyses can be performed using a recently developed MR method adapted from Egger regression (MR-Egger), which detects and explains directional pleiotropy in estimating causal factors [86].
5. Conclusions
In summary, HbA1c genetics can unveil new biological mechanisms in glucose metabolism, regulation, and perturbation, and T2D susceptibility. Given that HbA1c is a surrogate for overall ambient glycemia, HbA1c genetics provide complementary information to findings from genetic investigations on point-in-time measurements of glycemic traits such as FG.
Nonglycemic HbA1c genetic variants have important implications in clinical practice and public health, specifically HbA1c thresholds for T2D diagnosis, risk stratification, prediction, and the accuracy of lab assays.
The population impact of nonglycemic HbA1c genetic variants on measured HbA1c in screening for undiagnosed T2D may vary by ancestry. HbA1c genetics has challenged the traditional perspective of using HbA1c as a "one-size-fit-all" screening tool, and motivates research on individualized prevention strategies in this era of precision medicine.
Disclosures: The authors report no conflict of interests.
References
- Collins FS, Varmus H. A new initiative on precision medicine. N Engl J Med 2015. 372(9):793-795. [DOD] [CrossRef]
- Mullard A. $215 million precision-medicine initiative takes shape. Nat Rev Drug Discov 2015. 14:155. [DOD]
- American Diabetes Association. Diagnosis and classification of diabetes mellitus. Diabetes Care 2012. 35(Suppl 1):S64-S71. [DOD] [CrossRef]
- Mortensen HB, Christophersen C. Glucosylation of human haemoglobin a in red blood cells studied in vitro. Kinetics of the formation and dissociation of haemoglobin A1c. Clin Chim Acta 1983. 134(3):317-326. [DOD] [CrossRef]
- Simonis-Bik AM, Eekhoff EM, Diamant M, Boomsma DI, Heine RJ, Dekker JM, Willemsen G, van Leeuwen M, de Geus EJ. The heritability of HbA1c and fasting blood glucose in different measurement settings. Twin Res Hum Genet 2008. 11(6):597-602. [DOD] [CrossRef]
- Cohen RM, Snieder H, Lindsell CJ, Beyan H, Hawa MI, Blinko S, Edwards R, Spector TD, Leslie RD. Evidence for independent heritability of the glycation gap (glycosylation gap) fraction of HbA1c in nondiabetic twins. Diabetes Care 2006. 29(8):1739-1743. [DOD] [CrossRef]
- Carson AP, Reynolds K, Fonseca VA, Muntner P. Comparison of A1C and fasting glucose criteria to diagnose diabetes among U.S. adults. Diabetes Care 2010. 33(1):95-97. [DOD] [CrossRef]
- Cowie CC, Rust KF, Byrd-Holt DD, Gregg EW, Ford ES, Geiss LS, Bainbridge KE, Fradkin JE. Prevalence of diabetes and high risk for diabetes using A1C criteria in the U.S. population in 1988-2006. Diabetes Care 2010. 33(3):562-568. [DOD] [CrossRef]
- Pinelli NR, Jantz AS, Martin ET, Jaber LA. Sensitivity and specificity of glycated hemoglobin as a diagnostic test for diabetes and prediabetes in Arabs. J Clin Endocrinol Metab 2011. 96(10):E1680-E1683. [DOD] [CrossRef]
- Nakagami T, Tominaga M, Nishimura R, Yoshiike N, Daimon M, Oizumi T, Tajima N. Is the measurement of glycated hemoglobin A1c alone an efficient screening test for undiagnosed diabetes? Japan National Diabetes Survey. Diabetes Res Clin Pract 2007. 76(2):251-256. [DOD] [CrossRef]
- Panzer S, Kronik G, Lechner K, Bettelheim P, Neumann E, Dudczak R. Glycosylated hemoglobins (GHb): an index of red cell survival. Blood 1982. 59(6):1348-1350. [DOD]
- Sundaram RC, Selvaraj N, Vijayan G, Bobby Z, Hamide A, Rattina Dasse N. Increased plasma malondialdehyde and fructosamine in iron deficiency anemia: effect of treatment. Biomed Pharmacother 2007. 61(10):682-685. [DOD] [CrossRef]
- Coban E, Ozdogan M, Timuragaoglu A. Effect of iron deficiency anemia on the levels of hemoglobin A1c in nondiabetic patients. Acta Haematol 2004. 112(3):126-128. [DOD] [CrossRef]
- Little RR, Roberts WL. A review of variant hemoglobins interfering with hemoglobin A1c measurement. J Diabetes Sci Technol 2009. 3(3):446-451. [DOD] [CrossRef]
- Bry L, Chen PC, Sacks DB. Effects of hemoglobin variants and chemically modified derivatives on assays for glycohemoglobin. Clin Chem 2001. 47(2):153-163. [DOD]
- Pare G, Chasman DI, Parker AN, Nathan DM, Miletich JP, Zee RY, Ridker PM. Novel association of HK1 with glycated hemoglobin in a non-diabetic population: a genome-wide evaluation of 14,618 participants in the Women's Genome Health Study. Plos Genet 2008. 4(12):e1000312. [DOD] [CrossRef]
- Soranzo N, Sanna S, Wheeler E, Gieger C, Radke D, Dupuis J, Bouatia-Naji N, Langenberg C, Prokopenko I, Stolerman E, et al. Common variants at 10 genomic loci influence hemoglobin A(1)(C) levels via glycemic and nonglycemic pathways. Diabetes 2010. 59(12):3229-3239. [DOD] [CrossRef]
- Franklin CS, Aulchenko YS, Huffman JE, Vitart V, Hayward C, Polasek O, Knott S, Zgaga L, Zemunik T, Rudan I, et al. The TCF7L2 diabetes risk variant is associated with HbA(1)(C) levels: a genome-wide association meta-analysis. Ann Hum Genet 2010. 74(6):471-478. [DOD] [CrossRef]
- Ryu J, Lee C. Association of glycosylated hemoglobin with the gene encoding CDKAL1 in the Korean Association Resource (KARE) study. Hum Mutat 2012. 33(4):655-659. [DOD] [CrossRef]
- Chen P, Takeuchi F, Lee JY, Li H, Wu JY, Liang J, Long J, Tabara Y, Goodarzi MO, Pereira MA, et al. Multiple nonglycemic genomic loci are newly associated with blood level of glycated hemoglobin in East Asians. Diabetes 2014. 63(7):2551-2562. [DOD] [CrossRef]
- DIAbetes Genetics Replication And Meta-analysis (DIAGRAM) Consortium, Asian Genetic Epidemiology Network Type 2 Diabetes (AGEN-T2D) Consortium, South Asian Type 2 Diabetes (SAT2D) Consortium, Mexican American Type 2 Diabetes (MAT2D) Consortium, Type 2 Diabetes Genetic Exploration by Nex-generation sequencing in muylti-Ethnic Samples (T2D-GENES) Consortium, Mahajan A, Go MJ, Zhang W, Below JE, Gaulton KJ, et al. Genome-wide trans-ancestry meta-analysis provides insight into the genetic architecture of type 2 diabetes susceptibility. Nat Genet 2014. 46(3):234-244. [DOD] [CrossRef]
- Scott RA, Lagou V, Welch RP, Wheeler E, Montasser ME, Luan J, Magi R, Strawbridge RJ, Rehnberg E, Gustafsson S, et al. Large-scale association analyses identify new loci influencing glycemic traits and provide insight into the underlying biological pathways. Nat Genet 2012. 44(9):991-1005. [DOD] [CrossRef]
- Strawbridge RJ, Dupuis J, Prokopenko I, Barker A, Ahlqvist E, Rybin D, Petrie JR, Travers ME, Bouatia-Naji N, Dimas AS, et al. Genome-wide association identifies nine common variants associated with fasting proinsulin levels and provides new insights into the pathophysiology of type 2 diabetes. Diabetes 2011. 60(10):2624-2634. [DOD] [CrossRef]
- Jin T. The WNT signalling pathway and diabetes mellitus. Diabetologia 2008. 51(10):1771-1780. [DOD] [CrossRef]
- Billings LK, Jablonski KA, Ackerman RJ, Taylor A, Fanelli RR, McAteer JB, Guiducci C, Delahanty LM, Dabelea D, Kahn SE, et al. The influence of rare genetic variation in SLC30A8 on diabetes incidence and beta-cell function. J Clin Endocrinol Metab 2014. 99(5):E926-E930. [DOD] [CrossRef]
- Nicolson TJ, Bellomo EA, Wijesekara N, Loder MK, Baldwin JM, Gyulkhandanyan AV, Koshkin V, Tarasov AI, Carzaniga R, Kronenberger K, et al. Insulin storage and glucose homeostasis in mice null for the granule zinc transporter ZnT8 and studies of the type 2 diabetes-associated variants. Diabetes 2009. 58(9):2070-2083. [DOD] [CrossRef]
- Flannick J, Thorleifsson G, Beer NL, Jacobs SB, Grarup N, Burtt NP, Mahajan A, Fuchsberger C, Atzmon G, Benediktsson R, et al. Loss-of-function mutations in SLC30A8 protect against type 2 diabetes. Nat Genet 2014. 46(4):357-363. [DOD] [CrossRef]
- Prokopenko I, Langenberg C, Florez JC, Saxena R, Soranzo N, Thorleifsson G, Loos RJ, Manning AK, Jackson AU, Aulchenko Y, et al. Variants in MTNR1B influence fasting glucose levels. Nat Genet 2009. 41(1):77-81. [DOD] [CrossRef]
- Ramracheya RD, Muller DS, Squires PE, Brereton H, Sugden D, Huang GC, Amiel SA, Jones PM, Persaud SJ. Function and expression of melatonin receptors on human pancreatic islets. J Pineal Res 2008. 44(3):273-279. [DOD] [CrossRef]
- Matschinsky FM, Magnuson MA, Zelent D, Jetton TL, Doliba N, Han Y, Taub R, Grimsby J. The network of glucokinase-expressing cells in glucose homeostasis and the potential of glucokinase activators for diabetes therapy. Diabetes 2006. 55(1):1-12. [DOD] [CrossRef]
- Dimas AS, Lagou V, Barker A, Knowles JW, Magi R, Hivert MF, Benazzo A, Rybin D, Jackson AU, Stringham HM, et al. Impact of type 2 diabetes susceptibility variants on quantitative glycemic traits reveals mechanistic heterogeneity. Diabetes 2014. 63(6):2158-2171. [DOD] [CrossRef]
- Noe J, Stieger B, Meier PJ. Functional expression of the canalicular bile salt export pump of human liver. Gastroenterology 2002. 123(5):1659-1666. [DOD] [CrossRef]
- Adeva M, Gonzalez-Lucan M, Seco M, Donapetry C. Enzymes involved in l-lactate metabolism in humans. Mitochondrion 2013. 13(6):615-629. [DOD] [CrossRef]
- Murakami K, Kanno H, Tancabelic J, Fujii H. Gene expression and biological significance of hexokinase in erythroid cells. Acta Haematol 2002. 108(4):204-209. [DOD] [CrossRef]
- van Wijk R, Rijksen G, Huizinga EG, Nieuwenhuis HK, van Solinge WW. HK Utrecht: missense mutation in the active site of human hexokinase associated with hexokinase deficiency and severe nonspherocytic hemolytic anemia. Blood 2003. 101(1):345-347. [DOD] [CrossRef]
- Codd V, Mangino M, van der Harst P, Braund PS, Kaiser M, Beveridge AJ, Rafelt S, Moore J, Nelson C, Soranzo N, et al. Common variants near TERC are associated with mean telomere length. Nat Genet 2010. 42(3):197-199. [DOD] [CrossRef]
- Rubtsov AM, Lopina OD. Ankyrins. FEBS Lett 2000. 482(1-2):1-5. [DOD] [CrossRef]
- Gallagher PG, Nilson DG, Wong C, Weisbein JL, Garrett-Beal LJ, Eber SW, Bodine DM. A dinucleotide deletion in the ankyrin promoter alters gene expression, transcription initiation and TFIID complex formation in hereditary spherocytosis. Hum Mol Genet 2005. 14(17):2501-2509. [DOD] [CrossRef]
- van der Harst P, Zhang W, Mateo Leach I, Rendon A, Verweij N, Sehmi J, Paul DS, Elling U, Allayee H, Li X, et al. Seventy-five genetic loci influencing the human red blood cell. Nature 2012. 492(7429):369-375. [DOD] [CrossRef]
- Moltke I, Grarup N, Jorgensen ME, Bjerregaard P, Treebak JT, Fumagalli M, Korneliussen TS, Andersen MA, Nielsen TS, Krarup NT, et al. A common Greenlandic TBC1D4 variant confers muscle insulin resistance and type 2 diabetes. Nature 2014. 512(7513):190-193. [DOD] [CrossRef]
- Keaton JM, Cooke Bailey JN, Palmer ND, Freedman BI, Langefeld CD, Ng MC, Bowden DW. A comparison of type 2 diabetes risk allele load between African Americans and European Americans. Hum Genet 2014. 133(12):1487-1495. [DOD] [CrossRef]
- SIGMA Type 2 Diabetes Consortium, Estrada K, Aukrust I, Bjorkhaug L, Burtt NP, Mercader JM, Garcia-Ortiz H, Huerta-Chagoya A, Moreno-Macias H, Walford G, et al. Association of a low-frequency variant in HNF1A with type 2 diabetes in a Latino population. JAMA 2014. 311(22):2305-2314. [DOD] [CrossRef]
- Yang Q, Liu T, Shrader P, Yesupriya A, Chang MH, Dowling NF, Ned RM, Dupuis J, Florez JC, Khoury MJ, et al. Racial/ethnic differences in association of fasting glucose-associated genomic loci with fasting glucose, HOMA-B, and impaired fasting glucose in the U.S. adult population. Diabetes Care 2010. 33(11):2370-2377. [DOD] [CrossRef]
- Menzel S, Rooks H, Zelenika D, Mtatiro SN, Gnanakulasekaran A, Drasar E, Cox S, Liu L, Masood M, Silver N, et al. Global Genetic Architecture of an Erythroid Quantitative Trait Locus, HMIP-2. Ann Hum Genet 2014. 78(6):434-451. [DOD] [CrossRef]
- Lopez C, Saravia C, Gomez A, Hoebeke J, Patarroyo MA. Mechanisms of genetically-based resistance to malaria. Gene 2010. 467(1-2):1-12. [DOD] [CrossRef]
- Millimono TS, Loua KM, Rath SL, Relvas L, Bento C, Diakite M, Jarvis M, Daries N, Ribeiro LM, Manco L, Kaeda JS. High prevalence of hemoglobin disorders and glucose-6-phosphate dehydrogenase (G6PD) deficiency in the Republic of Guinea (West Africa). Hemoglobin 2012. 36(1):25-37. [DOD] [CrossRef]
- Maruthur NM, Kao WH, Clark JM, Brancati FL, Cheng CY, Pankow JS, Selvin E. Does genetic ancestry explain higher values of glycated hemoglobin in African Americans? Diabetes 2011. 60(9):2434-2438. [DOD]
- Selvin E, Parrinello CM, Sacks DB, Coresh J. Trends in prevalence and control of diabetes in the United States, 1988-1994 and 1999-2010. Ann Intern Med 2014. 160(8):517-525. [DOD] [CrossRef]
- Abdul-Ghani MA, Abdul-Ghani T, Muller G, Bergmann A, Fischer S, Bornstein S, Defronzo RA, Schwarz P. Role of glycated hemoglobin in the prediction of future risk of T2DM. J Clin Endocrinol Metab 2011. 96(8):2596-2600. [DOD] [CrossRef]
- Wannamethee SG, Papacosta O, Whincup PH, Thomas MC, Carson C, Lawlor DA, Ebrahim S, Sattar N. The potential for a two-stage diabetes risk algorithm combining non-laboratory-based scores with subsequent routine non-fasting blood tests: results from prospective studies in older men and women. Diabet Med 2011. 28(1):23-30. [DOD] [CrossRef]
- Norberg M, Eriksson JW, Lindahl B, Andersson C, Rolandsson O, Stenlund H, Weinehall L. A combination of HbA1c, fasting glucose and BMI is effective in screening for individuals at risk of future type 2 diabetes: OGTT is not needed. J Intern Med 2006. 260(3):263-271. [DOD] [CrossRef]
- Lim NK, Park SH, Choi SJ, Lee KS, Park HY. A risk score for predicting the incidence of type 2 diabetes in a middle-aged Korean cohort: the Korean genome and epidemiology study. Circ J 2012. 76(8):1904-1910. [DOD] [CrossRef]
- Sato KK, Hayashi T, Harita N, Yoneda T, Nakamura Y, Endo G, Kambe H. Combined measurement of fasting plasma glucose and A1C is effective for the prediction of type 2 diabetes: the Kansai Healthcare Study. Diabetes Care 2009. 32(4):644-646. [DOD] [CrossRef]
- Heianza Y, Arase Y, Hsieh SD, Saito K, Tsuji H, Kodama S, Tanaka S, Ohashi Y, Shimano H, Yamada N, et al. Development of a new scoring system for predicting the 5 year incidence of type 2 diabetes in Japan: the Toranomon Hospital Health Management Center Study 6 (TOPICS 6). Diabetologia 2012. 55(12):3213-3223. [DOD] [CrossRef]
- Choi SH, Kim TH, Lim S, Park KS, Jang HC, Cho NH. Hemoglobin A1c as a diagnostic tool for diabetes screening and new-onset diabetes prediction: a 6-year community-based prospective study. Diabetes Care 2011. 34(4):944-949. [DOD] [CrossRef]
- Bae JC, Rhee EJ, Lee WY, Park SE, Park CY, Oh KW, Park SW, Kim SW. Optimal range of HbA1c for the prediction of future diabetes: a 4-year longitudinal study. Diabetes Res Clin Pract 2011. 93(2):255-259. [DOD] [CrossRef]
- Valdes S, Botas P, Delgado E, Alvarez F, Diaz-Cadorniga F. HbA(1c) in the prediction of type 2 diabetes compared with fasting and 2-h post-challenge plasma glucose: The Asturias study (1998-2005). Diabetes Metab 2011. 37(1):27-32. [DOD] [CrossRef]
- Schottker B, Raum E, Rothenbacher D, Muller H, Brenner H. Prognostic value of haemoglobin A1c and fasting plasma glucose for incident diabetes and implications for screening. Eur J Epidemiol 2011. 26(10):779-787. [DOD] [CrossRef]
- Bonora E, Kiechl S, Mayr A, Zoppini G, Targher G, Bonadonna RC, Willeit J. High-normal HbA1c is a strong predictor of type 2 diabetes in the general population. Diabetes Care 2011. 34(4):1038-1040. [DOD] [CrossRef]
- Selvin E, Steffes MW, Gregg E, Brancati FL, Coresh J. Performance of A1C for the classification and prediction of diabetes. Diabetes Care 2011. 34(1):84-89. [DOD] [CrossRef]
- Kim DJ, Cho NH, Noh JH, Lee MS, Lee MK, Kim KW. Lack of excess maternal transmission of type 2 diabetes in a Korean population. Diabetes Res Clin Pract 2004. 65(2):117-124. [DOD] [CrossRef]
- The effect of intensive treatment of diabetes on the development and progression of long-term complications in insulin-dependent diabetes mellitus. The Diabetes Control and Complications Trial Research Group. N Engl J Med 1993. 329(14):977-986. [DOD] [CrossRef]
- Intensive blood-glucose control with sulphonylureas or insulin compared with conventional treatment and risk of complications in patients with type 2 diabetes (UKPDS 33). UK Prospective Diabetes Study (UKPDS) Group. Lancet 1998. 352(9131):837-853. [DOD] [CrossRef]
- Harmonizing HbA1c Testing - National Glycohemoglobin Standardization Program. http://www.ngsp.org/index.asp. [DOD]
- Use of Glycated Hemoglobin (HbA1c) in the Diagnosis of Diabetes Mellitus. http://www.who.int/diabetes/publications/diagnosis_diabetes2011/en/. [DOD]
- Canadian Diabetes Association Clinical Practice Guidelines Expert Committee, Goldenberg R, Punthakee Z. Definition, classification and diagnosis of diabetes, prediabetes and metabolic syndrome. Can J Diabetes 2013. 37(Suppl 1):S8-S11. [DOD]
- Li J, Ma H, Na L, Jiang S, Lv L, Li G, Zhang W, Na G, Li Y, Sun C. Increased Hemoglobin A1c threshold for prediabetes remarkably improving the agreement between A1c and oral glucose tolerance test criteria in obese population. J Clin Endocrinol Metab 2015. 100(5):1997-2005. [DOD] [CrossRef]
- Selvin E, Steffes MW, Zhu H, Matsushita K, Wagenknecht L, Pankow J, Coresh J, Brancati FL. Glycated hemoglobin, diabetes, and cardiovascular risk in nondiabetic adults. N Engl J Med 2010. 362(9):800-811. [DOD] [CrossRef]
- Sarwar N, Aspelund T, Eiriksdottir G, Gobin R, Seshasai SR, Forouhi NG, Sigurdsson G, Danesh J, Gudnason V. Markers of dysglycaemia and risk of coronary heart disease in people without diabetes: Reykjavik prospective study and systematic review. Plos Med 2010. 7(5):e1000278. [DOD] [CrossRef]
- Selvin E, Ning Y, Steffes MW, Bash LD, Klein R, Wong TY, Astor BC, Sharrett AR, Brancati FL, Coresh J. Glycated hemoglobin and the risk of kidney disease and retinopathy in adults with and without diabetes. Diabetes 2011. 60(1):298-305. [DOD] [CrossRef]
- Colagiuri S, Lee CM, Wong TY, Balkau B, Shaw JE, Borch-Johnsen K, DETECT-2 Collaboration Writing Group. Glycemic thresholds for diabetes-specific retinopathy: implications for diagnostic criteria for diabetes. Diabetes Care 2011. 34(1):145-150. [DOD] [CrossRef]
- International Expert Committee. International Expert Committee report on the role of the A1C assay in the diagnosis of diabetes. Diabetes Care 2009. 32(7):1327-1334. [DOD] [CrossRef]
- Zhang X, Gregg EW, Williamson DF, Barker LE, Thomas W, Bullard KM, Imperatore G, Williams DE, Albright AL. A1C level and future risk of diabetes: a systematic review. Diabetes Care 2010. 33(7):1665-1673. [DOD] [CrossRef]
- Holman RR, Paul SK, Bethel MA, Matthews DR, Neil HA. 10-year follow-up of intensive glucose control in type 2 diabetes. N Engl J Med 2008. 359(15):1577-1589. [DOD] [CrossRef]
- Action to Control Cardiovascular Risk in Diabetes Study Group, Gerstein HC, Miller ME, Byington RP, Goff DC Jr, Bigger JT, Buse JB, Cushman WC, Genuth S, Ismail-Beigi F, et al. Effects of intensive glucose lowering in type 2 diabetes. N Engl J Med 2008. 358(24):2545-2559. [DOD] [CrossRef]
- ACCORD Study Group, Gerstein HC, Miller ME, Genuth S, Ismail-Beigi F, Buse JB, Goff DC Jr, Probstfield JL, Cushman WC, Ginsberg HN, et al. Long-term effects of intensive glucose lowering on cardiovascular outcomes. N Engl J Med 2011. 364(9):818-828. [DOD] [CrossRef]
- ADVANCE Collaborative Group, Patel A, MacMahon S, Chalmers J, Neal B, Billot L, Woodward M, Marre M, Cooper M, Glasziou P, et al. Intensive blood glucose control and vascular outcomes in patients with type 2 diabetes. N Engl J Med 2008. 358(24):2560-2572. [DOD] [CrossRef]
- Duckworth W, Abraira C, Moritz T, Reda D, Emanuele N, Reaven PD, Zieve FJ, Marks J, Davis SN, Hayward R, et al. Glucose control and vascular complications in veterans with type 2 diabetes. N Engl J Med 2009. 360(2):129-139. [DOD] [CrossRef]
- Investigators OT, Mellbin LG, Ryden L, Riddle MC, Probstfield J, Rosenstock J, Diaz R, Yusuf S, Gerstein HC. Does hypoglycaemia increase the risk of cardiovascular events? A report from the ORIGIN trial. Eur Heart J 2013. 34(40):3137-3144. [DOD] [CrossRef]
- Investigators OT, Gerstein HC, Bosch J, Dagenais GR, Diaz R, Jung H, Maggioni AP, Pogue J, Probstfield J, Ramachandran A, et al. Basal insulin and cardiovascular and other outcomes in dysglycemia. N Engl J Med 2012. 367(4):319-328. [DOD] [CrossRef]
- Lawlor DA, Harbord RM, Sterne JA, Timpson N, Davey Smith G. Mendelian randomization: using genes as instruments for making causal inferences in epidemiology. Stat Med 2008. 27(8):1133-1163. [DOD] [CrossRef]
- Palmer TM, Lawlor DA, Harbord RM, Sheehan NA, Tobias JH, Timpson NJ, Davey Smith G, Sterne JA. Using multiple genetic variants as instrumental variables for modifiable risk factors. Stat Methods Med Res 2012. 21(3):223-242. [DOD] [CrossRef]
- Burgess S, Butterworth A, Thompson SG. Mendelian randomization analysis with multiple genetic variants using summarized data. Genet Epidemiol 2013. 37(7):658-665. [DOD] [CrossRef]
- Burgess S, Thompson SG. Use of allele scores as instrumental variables for Mendelian randomization. Int J Epidemiol 2013. 42(4):1134-1144. [DOD] [CrossRef]
- Glymour MM, Tchetgen Tchetgen EJ, Robins JM. Credible Mendelian randomization studies: approaches for evaluating the instrumental variable assumptions. Am J Epidemiol 2012. 175(4):332-339. [DOD] [CrossRef]
- Bowden J, Davey Smith G, Burgess S. Mendelian randomization with invalid instruments: effect estimation and bias detection through Egger regression. Int J Epidemiol 2015. 44(2):512-525. [DOD] [CrossRef]
- Roder ME, Porte D Jr, Schwartz RS, Kahn SE. Disproportionately elevated proinsulin levels reflect the degree of impaired B cell secretory capacity in patients with noninsulin-dependent diabetes mellitus. J Clin Endocrinol Metab 1998. 83(2):604-608. [DOD]
- Wareham NJ, Byrne CD, Williams R, Day NE, Hales CN. Fasting proinsulin concentrations predict the development of type 2 diabetes. Diabetes Care 1999. 22(2):262-270. [DOD] [CrossRef]
This article has been cited by other articles:
|